Regression Of X On Y Or Y On X
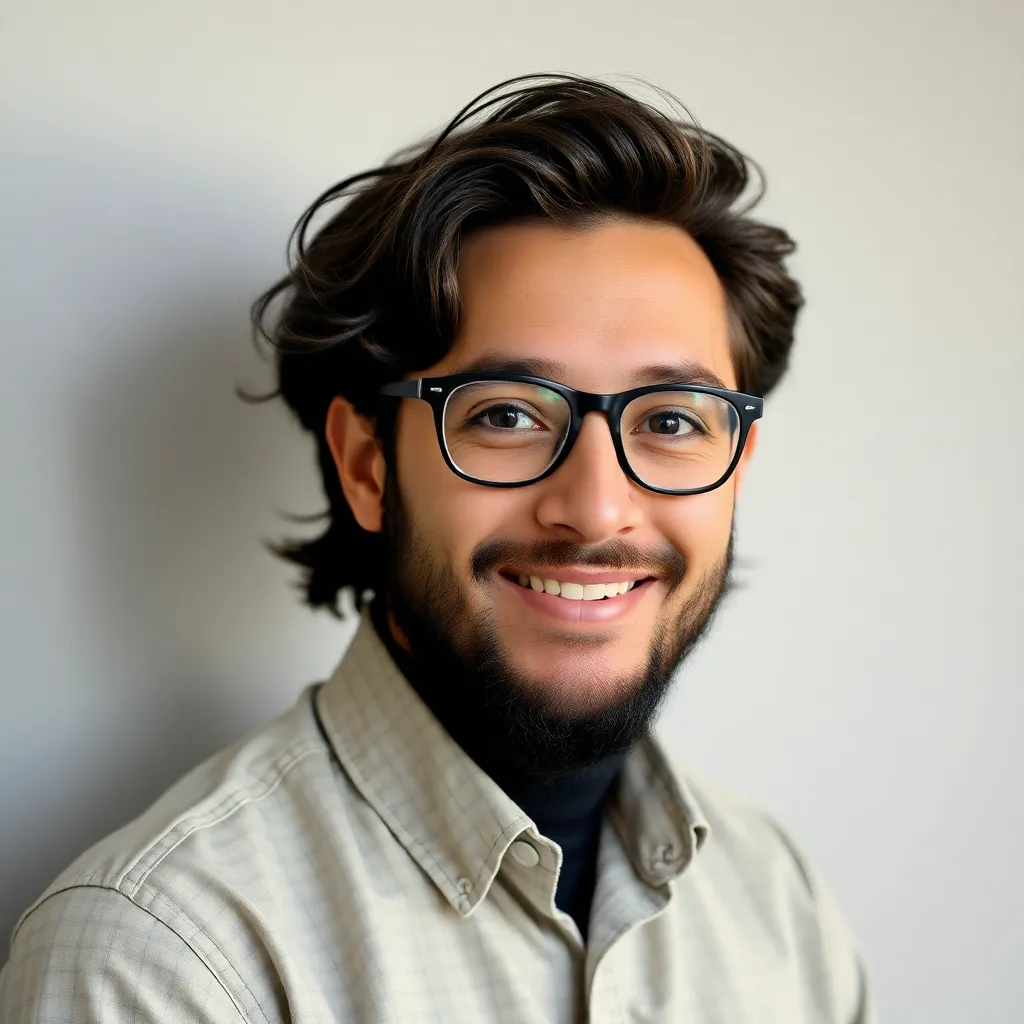
News Leon
Apr 18, 2025 · 6 min read

Table of Contents
Regression of X on Y or Y on X: Unveiling the Subtleties of Statistical Relationships
Regression analysis is a cornerstone of statistical modeling, allowing us to explore and quantify the relationships between variables. A common question that arises, especially for beginners, is the distinction between regressing X on Y versus regressing Y on X. While seemingly a simple swap, this choice has significant implications for the interpretation and application of the results. This comprehensive guide delves into the nuances of this difference, illuminating the practical considerations and theoretical underpinnings.
Understanding the Basics of Linear Regression
Before diving into the X on Y vs. Y on X debate, let's establish a firm grasp on the fundamentals of linear regression. In its simplest form, linear regression models the relationship between a dependent variable (often denoted as Y) and one or more independent variables (often denoted as X). The model assumes a linear relationship, meaning the change in Y is proportional to the change in X. The goal is to find the "best-fitting" line that minimizes the sum of squared differences between the observed Y values and the values predicted by the model. This "best-fitting" line is defined by its slope and intercept.
The Regression Equation: Deciphering the Coefficients
The standard linear regression equation is represented as:
Y = β₀ + β₁X + ε
Where:
- Y is the dependent variable.
- X is the independent variable.
- β₀ is the y-intercept (the value of Y when X = 0).
- β₁ is the slope (the change in Y for a one-unit change in X).
- ε is the error term (representing the unexplained variation).
This equation forms the basis for understanding the relationship between X and Y. The coefficients, β₀ and β₁, are estimated from the data using statistical methods such as ordinary least squares (OLS).
Regression of X on Y: A Different Perspective
When we regress X on Y, we essentially flip the roles of the dependent and independent variables. The equation becomes:
X = β₀ + β₁Y + ε
Now, Y is the predictor variable, and X is the response variable. This seemingly simple change has profound implications for the interpretation of the results. The slope (β₁) now represents the change in X for a one-unit change in Y. The context and interpretation of the model are entirely different.
Implications of Switching Variables
The key difference lies in the causal inference. Regressing Y on X implies that X influences Y, suggesting a directional relationship where changes in X cause changes in Y. However, regressing X on Y suggests a different causal interpretation: Y influences X. This reversal of causality is crucial and often overlooked.
Furthermore, the coefficients, R-squared, and other statistical measures will differ significantly between the two regressions. The best-fitting line will be different, reflecting the differing roles of X and Y. This is especially important when dealing with datasets where the correlation between X and Y is not perfect (i.e., less than 1). The regression line in each case will represent the line of best fit given the specific roles of X and Y.
Practical Examples Illustrating the Distinction
Let's consider a few scenarios to further clarify the implications of choosing between regressing X on Y and Y on X:
Scenario 1: Height and Weight
Imagine analyzing the relationship between height (X) and weight (Y) in a population.
-
Y on X: Regressing weight (Y) on height (X) implies that height influences weight. This is a reasonable assumption, as taller individuals tend to weigh more. The slope would represent the increase in weight for each unit increase in height.
-
X on Y: Regressing height (X) on weight (Y) implies that weight influences height. This is less intuitive and likely less accurate. While weight can influence height in extreme cases, it's not the primary driver of height. The slope would represent the increase in height for each unit increase in weight.
Scenario 2: Advertising Spend and Sales
Consider the relationship between advertising expenditure (X) and sales revenue (Y).
-
Y on X: Regressing sales (Y) on advertising spend (X) suggests that advertising expenditure influences sales. This is a common marketing analysis, where the goal is to determine the return on advertising investment.
-
X on Y: Regressing advertising spend (X) on sales (Y) would imply that sales influence advertising expenditure. This suggests that companies increase advertising spending in response to higher sales. This is also a plausible scenario, reflecting a company's adjustment of marketing budgets based on sales performance.
Scenario 3: Temperature and Ice Cream Sales
Analyze the relationship between daily temperature (X) and daily ice cream sales (Y).
-
Y on X: Regressing ice cream sales (Y) on temperature (X) suggests that temperature influences ice cream sales. This is logical, as higher temperatures usually lead to increased ice cream consumption.
-
X on Y: Regressing temperature (X) on ice cream sales (Y) implies that ice cream sales influence temperature. This is nonsensical. Temperature is not influenced by ice cream sales.
Beyond Simple Linear Regression: Extending the Concepts
The distinction between regressing X on Y and Y on X extends beyond simple linear regression to more complex models, including multiple linear regression and non-linear models. The core concept remains the same: the choice of dependent and independent variables significantly impacts the interpretation and implications of the results. In multiple regression, where multiple independent variables are included, selecting the appropriate dependent variable is crucial for accurately modeling the relationships and making valid causal inferences.
Addressing Potential Pitfalls and Misinterpretations
Several common pitfalls and misinterpretations should be avoided when dealing with X on Y versus Y on X regressions:
-
Causation vs. Correlation: Regression analysis only reveals correlations, not causations. Even if a strong relationship is found, it doesn't necessarily imply causality. Other factors may be influencing the relationship.
-
Reverse Causality: Carefully consider the possibility of reverse causality. It's essential to consider which variable is the likely cause and which is the likely effect. The wrong choice can lead to misleading conclusions.
-
Overfitting: Overfitting the model to the specific dataset can lead to inaccurate predictions on new data. Careful model selection and validation are crucial to avoid this.
-
Ignoring Confounding Variables: Always consider the possibility of confounding variables—variables that affect both X and Y, potentially distorting the observed relationship. Techniques such as multivariate analysis can help to control for these variables.
Conclusion: The Importance of Context and Careful Interpretation
The choice between regressing X on Y or Y on X is not arbitrary. It is a crucial decision that depends heavily on the research question, the underlying context, and the causal relationships being investigated. Carefully considering the implications of this choice, understanding the limitations of regression analysis, and avoiding common pitfalls are essential for generating accurate, reliable, and meaningful statistical insights. The selection of the appropriate regression model should always be driven by a thorough understanding of the variables and their potential relationships, along with a clear definition of the research objectives. By thoughtfully approaching this decision, researchers can leverage regression analysis to extract valuable insights and contribute to a deeper understanding of the phenomena under study. Remember to always validate your results with multiple approaches and contextual knowledge, ensuring that your analysis accurately reflects the complexities of the real-world relationships being examined.
Latest Posts
Latest Posts
-
The Product Of Two Consecutive Even Integers Is 288
Apr 19, 2025
-
Identify The Following Salts As Acidic Basic Or Neutral
Apr 19, 2025
-
Which Of The Following Is Not A Consumer
Apr 19, 2025
-
Which Of The Following Is Not An Optical Storage Device
Apr 19, 2025
-
The Mean Of A Sample Is
Apr 19, 2025
Related Post
Thank you for visiting our website which covers about Regression Of X On Y Or Y On X . We hope the information provided has been useful to you. Feel free to contact us if you have any questions or need further assistance. See you next time and don't miss to bookmark.