The Mean Of A Sample Is
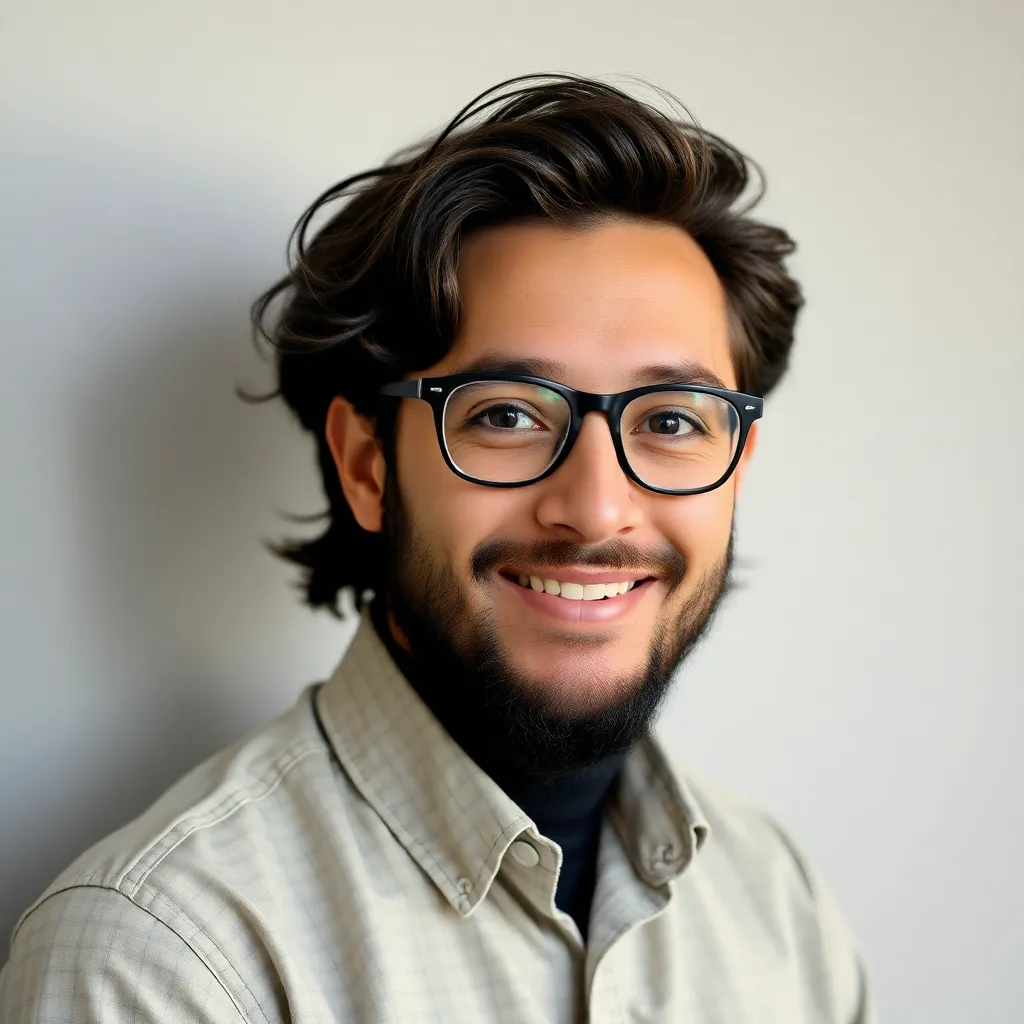
News Leon
Apr 19, 2025 · 7 min read

Table of Contents
The Mean of a Sample: A Deep Dive into Statistical Significance
The mean of a sample is a fundamental concept in statistics, forming the bedrock of numerous analytical techniques. Understanding its properties, limitations, and applications is crucial for anyone working with data, from students analyzing experimental results to data scientists building predictive models. This comprehensive guide will explore the mean of a sample, delving into its calculation, interpretation, and significance in statistical inference.
What is the Mean of a Sample?
The mean of a sample, often referred to as the sample mean, is the average of a set of observations drawn from a larger population. It's a descriptive statistic that provides a single value summarizing the central tendency of the sample data. Unlike the population mean (μ), which represents the true average of the entire population, the sample mean (x̄) is an estimate based on a subset of that population. This distinction is vital, as the sample mean is subject to sampling variability – it can fluctuate depending on the specific sample selected.
Calculating the Sample Mean
Calculating the sample mean is straightforward. For a sample of size 'n', containing observations x₁, x₂, ..., xₙ, the formula for the sample mean is:
x̄ = (Σxᵢ) / n
Where:
- x̄ represents the sample mean
- Σxᵢ represents the sum of all observations in the sample
- n represents the sample size
Let's illustrate this with an example. Consider a sample of five exam scores: 85, 92, 78, 88, and 90. The sample mean is calculated as follows:
x̄ = (85 + 92 + 78 + 88 + 90) / 5 = 86.6
Therefore, the sample mean exam score is 86.6.
The Importance of Sample Mean in Statistical Inference
The sample mean plays a pivotal role in statistical inference, which involves drawing conclusions about a population based on sample data. Its importance stems from its properties as an estimator of the population mean:
1. Unbiased Estimator
The sample mean is an unbiased estimator of the population mean. This means that, on average, the sample mean will equal the population mean over many repeated samples. While a single sample mean might differ from the population mean due to random sampling error, the average of many sample means will converge towards the true population mean.
2. Efficiency
The sample mean is also a relatively efficient estimator. This implies that it has a smaller variance (spread) compared to other estimators of the population mean. A smaller variance translates to greater precision in estimating the population mean. In simpler terms, the sample mean tends to be closer to the true population mean than other potential estimators.
3. Central Limit Theorem
The Central Limit Theorem (CLT) is a cornerstone of statistical inference and directly relates to the sample mean. The CLT states that the distribution of sample means from a large number of independent, identically distributed random samples will approximate a normal distribution, regardless of the shape of the underlying population distribution. This holds true even if the original population distribution is not normal, provided the sample size is sufficiently large (generally considered to be n ≥ 30). This property allows us to use the normal distribution to make inferences about the population mean, even when we don't know the population distribution.
Sampling Distribution of the Sample Mean
The concept of the sampling distribution is crucial for understanding how the sample mean relates to the population mean. The sampling distribution of the sample mean is the probability distribution of all possible sample means that could be obtained from a population. This distribution is characterized by its mean and standard deviation.
Mean of the Sampling Distribution
The mean of the sampling distribution of the sample mean is equal to the population mean (μ). This reinforces the unbiased nature of the sample mean as an estimator.
Standard Error of the Mean
The standard deviation of the sampling distribution of the sample mean is known as the standard error of the mean (SEM). It measures the variability of the sample means around the population mean. The formula for the SEM is:
SEM = σ / √n
Where:
- σ is the population standard deviation
- n is the sample size
The SEM is inversely proportional to the square root of the sample size. This means that as the sample size increases, the SEM decreases. A smaller SEM indicates that the sample means are clustered more tightly around the population mean, leading to a more precise estimate. If the population standard deviation (σ) is unknown, the sample standard deviation (s) is often used as an estimate, leading to the following formula:
SEM ≈ s / √n
Applications of the Sample Mean
The sample mean finds extensive applications across various fields:
1. Hypothesis Testing
The sample mean is a crucial element in hypothesis testing, a statistical procedure used to determine whether there's sufficient evidence to reject a null hypothesis. For example, in a clinical trial comparing two treatments, the sample means of the outcome variable (e.g., blood pressure) for each treatment group are compared to determine if there's a statistically significant difference between the treatments.
2. Confidence Intervals
Confidence intervals provide a range of values within which the population mean is likely to fall with a certain level of confidence. The sample mean is the center of the confidence interval, and the width of the interval is determined by the SEM and the chosen confidence level (e.g., 95%).
3. Regression Analysis
In regression analysis, the sample means of the dependent and independent variables are used to estimate the regression line, which models the relationship between the variables.
4. Quality Control
In quality control, the sample mean is used to monitor the average value of a product characteristic (e.g., weight, length) to ensure that it meets predetermined specifications.
5. Data Visualization
The sample mean is often displayed in graphs and charts (like histograms and box plots) to provide a visual representation of the central tendency of the data.
Limitations of the Sample Mean
While the sample mean is a powerful tool, it's essential to be aware of its limitations:
1. Sensitivity to Outliers
The sample mean is highly sensitive to outliers – extreme values that deviate significantly from the rest of the data. Outliers can disproportionately influence the sample mean, leading to a biased estimate of the population mean. Robust measures of central tendency, such as the median, are less affected by outliers.
2. Not Suitable for All Data Types
The sample mean is primarily suitable for numerical data that are measured on an interval or ratio scale. It's not appropriate for nominal or ordinal data, which represent categories or ranks rather than numerical values.
3. Assumption of Random Sampling
The validity of inferences based on the sample mean relies on the assumption that the sample is randomly selected from the population. If the sample is biased (e.g., not representative of the population), the sample mean will not accurately estimate the population mean.
Choosing the Right Measure of Central Tendency
The decision of whether to use the sample mean or other measures of central tendency depends on the nature of the data and the research question. Here's a brief comparison:
- Mean: Suitable for numerical data, sensitive to outliers, provides a precise estimate of the central tendency for symmetric distributions.
- Median: Suitable for numerical data, less sensitive to outliers than the mean, a better representation of central tendency for skewed distributions.
- Mode: Suitable for all data types, represents the most frequent value in a dataset, useful for identifying the most common category or value.
Conclusion
The sample mean is a fundamental concept in statistics with wide-ranging applications. Its properties as an unbiased and efficient estimator of the population mean, coupled with the power of the Central Limit Theorem, make it an indispensable tool for statistical inference. However, it's crucial to acknowledge its limitations, particularly its sensitivity to outliers and the importance of random sampling. By understanding the strengths and weaknesses of the sample mean and considering alternative measures of central tendency, data analysts can make informed decisions and draw meaningful conclusions from their data. Remember that the appropriate measure of central tendency depends on the specific context and the nature of the data under consideration. Careful consideration of these factors will lead to more robust and reliable analyses.
Latest Posts
Latest Posts
-
Which Organ Converts Ammonia To Urea
Apr 20, 2025
-
A Student Crazed By Final Exams
Apr 20, 2025
-
Dna Replication Occurs In Which Phase Of Meiosis
Apr 20, 2025
-
Find The Value Of X In The Isosceles Triangle
Apr 20, 2025
-
0 25 Mole Of Mg Contains How Many Atoms
Apr 20, 2025
Related Post
Thank you for visiting our website which covers about The Mean Of A Sample Is . We hope the information provided has been useful to you. Feel free to contact us if you have any questions or need further assistance. See you next time and don't miss to bookmark.