Most Stable Measure Of Central Tendency
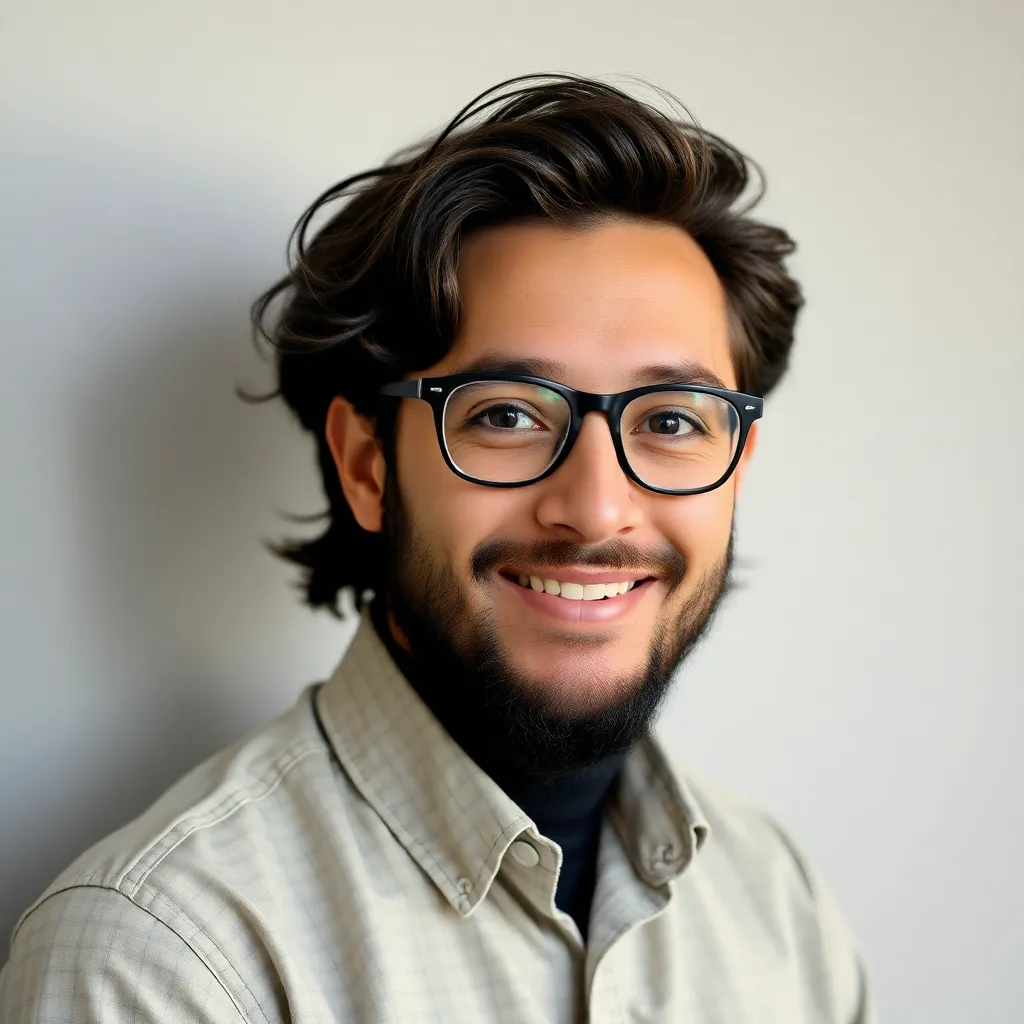
News Leon
Mar 25, 2025 · 6 min read
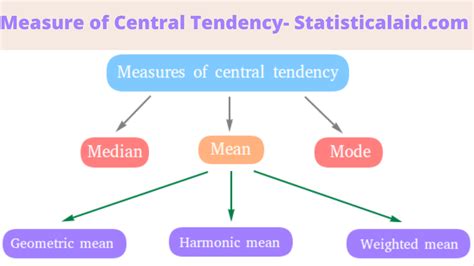
Table of Contents
- Most Stable Measure Of Central Tendency
- Table of Contents
- The Most Stable Measure of Central Tendency: A Deep Dive into Robust Statistics
- Understanding Central Tendency: Mean, Median, and Mode
- The Mean (Average)
- The Median
- The Mode
- Stability and the Impact of Outliers
- Mean's Instability: A Vulnerability to Outliers and Skewness
- Median's Robustness: Stability in the Face of Outliers and Skewness
- Mode's Limitations: Suitability for Specific Data Types
- Robustness and its Significance in Statistical Analysis
- Practical Applications: When to Choose the Median
- Beyond the Median: Other Robust Measures
- Conclusion: The Median as a Foundation of Stable Data Analysis
- Latest Posts
- Latest Posts
- Related Post
The Most Stable Measure of Central Tendency: A Deep Dive into Robust Statistics
Choosing the right measure of central tendency is crucial for accurate data representation and insightful analysis. While the mean, median, and mode each offer unique perspectives on the "center" of a dataset, their stability—their resistance to outliers and skewed distributions—varies significantly. This article explores the concept of stability in central tendency measures, highlighting why robust statistics, particularly the median, often emerges as the most stable option. We will delve into the properties of each measure, examine their behavior under different conditions, and demonstrate why understanding stability is paramount for reliable data interpretation.
Understanding Central Tendency: Mean, Median, and Mode
Before exploring stability, let's briefly review the three primary measures of central tendency:
The Mean (Average)
The mean is calculated by summing all values in a dataset and dividing by the number of values. It's widely used and easily understood, providing a straightforward representation of the average value. However, its sensitivity to outliers is a significant drawback. A single extremely high or low value can drastically skew the mean, misrepresenting the true center of the data.
Example: Consider the dataset: {2, 3, 4, 5, 100}. The mean is 22.8, heavily influenced by the outlier (100). This mean doesn't accurately reflect the central tendency of the majority of the data points.
The Median
The median represents the middle value in a dataset when the data is ordered. It's less sensitive to outliers than the mean because extreme values don't directly influence its calculation. For datasets with an even number of values, the median is the average of the two middle values.
Example: Using the same dataset {2, 3, 4, 5, 100}, the median is 4. This provides a far more accurate representation of the central tendency than the mean in this case.
The Mode
The mode is the value that appears most frequently in a dataset. It's useful for categorical data and datasets with multiple peaks (multimodal distributions). However, it might not exist (no repeated values) or multiple modes can exist, making it less reliable as a single measure of central tendency compared to the median and mean.
Example: In the dataset {1, 2, 2, 3, 4, 4, 4, 5}, the mode is 4.
Stability and the Impact of Outliers
The stability of a central tendency measure refers to its resistance to changes caused by outliers or deviations from a normal distribution. Outliers are extreme values that significantly differ from the majority of the data. Skewness refers to the asymmetry of a distribution, indicating a concentration of data points on one side of the mean.
Outliers and Skewness: Outliers disproportionately affect the mean, pulling it towards the extreme values. Skewed distributions also influence the mean, while the median remains relatively unaffected.
Mean's Instability: A Vulnerability to Outliers and Skewness
The mean is highly sensitive to both outliers and skewness. A single outlier can dramatically shift the mean, providing a misleading representation of the central tendency. Similarly, in skewed distributions, the mean is pulled towards the tail of the distribution, deviating from the typical value. This instability makes the mean unreliable when dealing with data containing outliers or significant skewness.
Median's Robustness: Stability in the Face of Outliers and Skewness
The median's key advantage lies in its robustness. It's far less affected by outliers than the mean. Extreme values do not directly influence the median's calculation; they only affect its position in the ordered dataset. In skewed distributions, the median provides a more stable and representative measure of the central tendency than the mean. This is because it's not sensitive to the extreme values that characterize skewed data.
Mode's Limitations: Suitability for Specific Data Types
The mode, while useful for identifying the most frequent value, is generally less stable than the median. Its value is not affected by outliers, but its existence and uniqueness are dependent on the data's distribution. Multiple modes can exist, making it difficult to identify a single representative central tendency. The mode is also not suited for continuous data.
Robustness and its Significance in Statistical Analysis
Robustness is a crucial property in statistical analysis, particularly when dealing with real-world datasets. These datasets often contain errors, outliers, or deviations from the assumptions of typical statistical methods. Robust statistics, methods that are less sensitive to violations of assumptions, are essential for drawing reliable conclusions. The median, due to its resistance to outliers, is a cornerstone of robust statistics.
Advantages of using robust statistics:
- Reliable Results: Robust statistics provide reliable results even when data contains outliers or deviates from normality assumptions.
- Accurate Representation: They give a more accurate representation of the central tendency in real-world, imperfect datasets.
- Reduced Bias: They minimize the influence of extreme values, reducing bias in the results.
- Increased Confidence: Using robust statistics increases confidence in the findings and their interpretations.
Practical Applications: When to Choose the Median
The choice of central tendency measure depends heavily on the data's characteristics and the research question. The median shines in situations where robustness is critical:
- Income Data: Income data often contains outliers (extremely high incomes). The median income provides a more accurate reflection of the typical income than the mean, which can be significantly inflated by a few high earners.
- Real Estate Prices: Similar to income data, real estate prices can exhibit outliers (very expensive properties). The median price is a better representation of the typical market value.
- Environmental Monitoring: Environmental data may contain outliers due to measurement errors or unusual events. The median provides a more stable representation of typical environmental conditions.
- Clinical Trials: In clinical trials, outlier results might stem from individual patient responses. The median response may better reflect the overall treatment effect than the mean.
- Exploratory Data Analysis (EDA): Before applying more complex statistical methods, the median can help provide initial insights into the dataset's central tendency, particularly its resistance to outliers.
Beyond the Median: Other Robust Measures
While the median is a highly robust measure, other robust statistical methods further enhance the stability of analyses. These include:
- Trimmed Mean: This is calculated by removing a certain percentage of the highest and lowest values from a dataset before calculating the mean. This reduces the influence of outliers.
- Winsorized Mean: Similar to the trimmed mean, this method replaces extreme values with less extreme values (often the highest and lowest values are replaced with the next highest and lowest values) before calculating the mean.
- M-estimators: These are a class of robust estimators of location, designed to be resistant to outliers. They are more complex than the median but offer even greater robustness.
Conclusion: The Median as a Foundation of Stable Data Analysis
In conclusion, while the mean, median, and mode each serve valuable purposes in describing data, the median stands out as the most stable measure of central tendency. Its resistance to outliers and skewness makes it a cornerstone of robust statistics, offering more reliable and accurate representations of central tendency in real-world data sets. Understanding the stability of different central tendency measures is vital for effective data analysis, leading to more accurate interpretations and informed decision-making. By employing robust statistical methods, such as prioritizing the median, analysts can mitigate the impact of problematic data points and ensure the reliability of their findings. Choosing the appropriate measure depends heavily on the specific context and the goals of the analysis, but for situations where stability and resilience to outliers are paramount, the median emerges as the clear winner.
Latest Posts
Latest Posts
-
What Will Happen If Ribosomes Are Removed From The Cell
Mar 29, 2025
-
How Many Hydrogen Bonds Between C And G
Mar 29, 2025
-
Regulates What Enters And Leaves The Cell
Mar 29, 2025
-
The Most Active Phagocytic Cells In Circulating Blood Are
Mar 29, 2025
-
How Many Cups In 9 Ounces
Mar 29, 2025
Related Post
Thank you for visiting our website which covers about Most Stable Measure Of Central Tendency . We hope the information provided has been useful to you. Feel free to contact us if you have any questions or need further assistance. See you next time and don't miss to bookmark.