Independent Variable Dependent Variable Control Variable
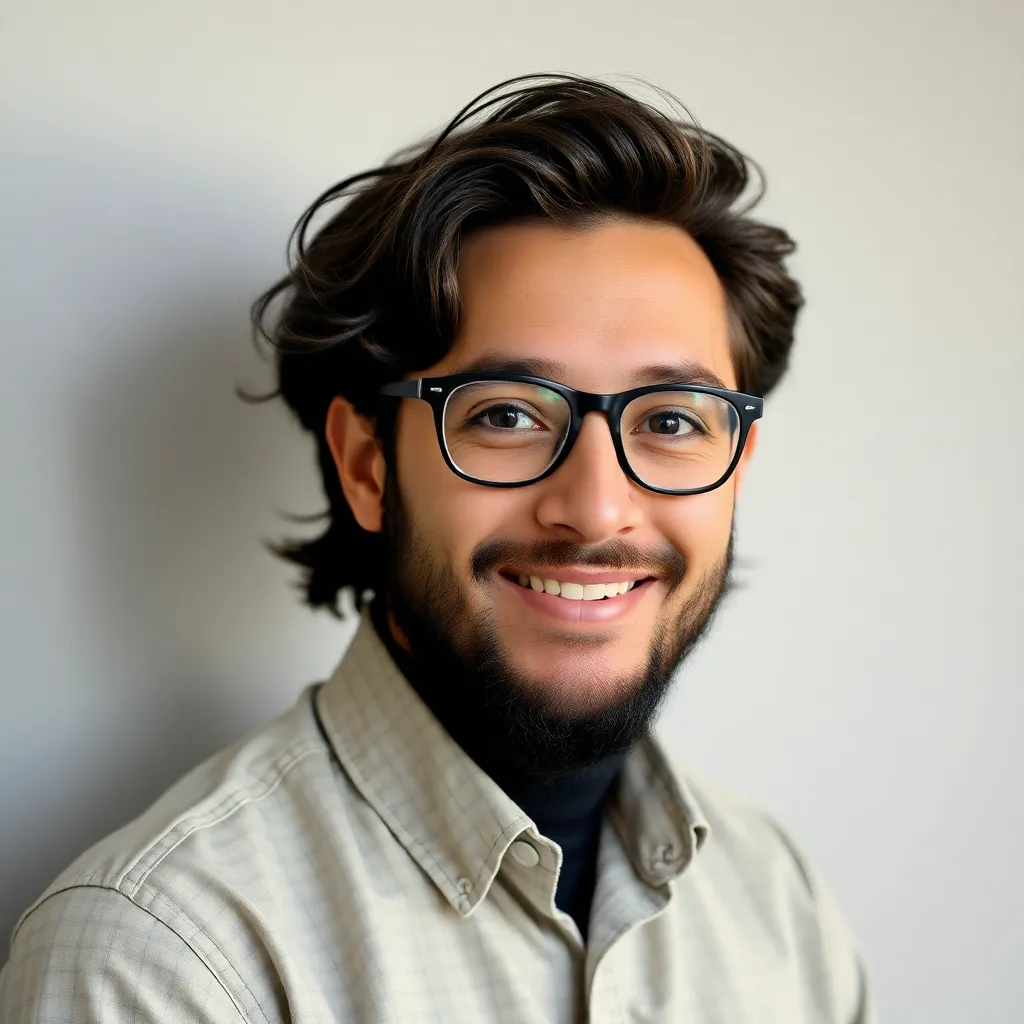
News Leon
Apr 06, 2025 · 6 min read
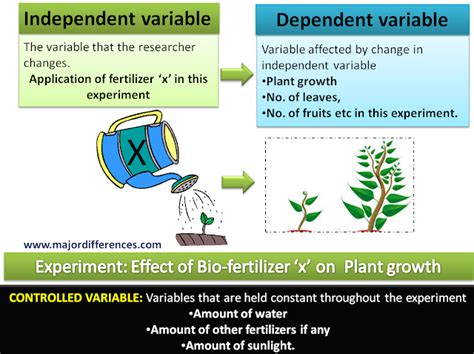
Table of Contents
Understanding Independent, Dependent, and Control Variables: A Comprehensive Guide
In the world of research and experimentation, understanding the roles of independent, dependent, and control variables is crucial for conducting meaningful and reliable studies. These variables are the building blocks of any scientific investigation, forming the foundation upon which conclusions are drawn and hypotheses are tested. This comprehensive guide delves into each variable type, providing clear definitions, practical examples, and strategies for effective implementation in your research.
What is an Independent Variable?
The independent variable (IV) is the variable that is manipulated or changed by the researcher. It's the factor that is believed to cause an effect or change in another variable. Think of it as the cause in a cause-and-effect relationship. The researcher carefully controls and alters the independent variable to observe its impact on the dependent variable. This manipulation is a key element differentiating experimental research from other research methods.
Identifying the Independent Variable
Identifying the independent variable often involves asking: "What am I changing or manipulating in my experiment?" The answer to this question usually pinpoints the independent variable. It's the variable that the researcher has direct control over.
Examples of Independent Variables:
- In a study on the effect of fertilizer on plant growth: The type or amount of fertilizer is the independent variable.
- In an experiment examining the impact of sleep deprivation on cognitive performance: The amount of sleep deprivation (e.g., 4 hours, 6 hours, 8 hours) is the independent variable.
- In a study exploring the effectiveness of different teaching methods on student learning: The teaching method (e.g., lecture, group work, online learning) is the independent variable.
What is a Dependent Variable?
The dependent variable (DV) is the variable that is measured or observed. It's the variable that is affected by the changes in the independent variable. Think of it as the effect in a cause-and-effect relationship. The researcher observes how the dependent variable changes in response to the manipulation of the independent variable.
Identifying the Dependent Variable
Identifying the dependent variable involves asking: "What am I measuring or observing?" The answer usually indicates the dependent variable. It's the variable that is expected to change because of the manipulation of the independent variable. The dependent variable is dependent on the independent variable.
Examples of Dependent Variables:
- In a study on the effect of fertilizer on plant growth: The height or weight of the plants is the dependent variable.
- In an experiment examining the impact of sleep deprivation on cognitive performance: Performance on cognitive tests (e.g., reaction time, memory recall) is the dependent variable.
- In a study exploring the effectiveness of different teaching methods on student learning: Student test scores or grades are the dependent variable.
What is a Control Variable?
Control variables (also known as constant variables or extraneous variables) are variables that are kept constant or controlled throughout the experiment. They are factors that could potentially influence the dependent variable, but the researcher wants to eliminate their influence to isolate the effect of the independent variable. Controlling these variables ensures that any observed changes in the dependent variable are truly due to the manipulation of the independent variable, and not due to other confounding factors.
The Importance of Control Variables
Control variables are crucial for establishing internal validity. Internal validity refers to the confidence that the observed effects are due to the independent variable and not other factors. Without proper control of extraneous variables, the researcher cannot confidently conclude that the independent variable caused the observed changes in the dependent variable.
Identifying and Controlling Variables
Identifying potential control variables requires careful consideration of all factors that could influence the dependent variable. The researcher then implements strategies to keep these variables constant across all experimental groups. This might involve using standardized procedures, matching participants across groups based on relevant characteristics, or using random assignment to distribute extraneous variables evenly.
Examples of Control Variables:
- In a study on the effect of fertilizer on plant growth: The amount of sunlight, water, and soil type would be control variables. These factors could affect plant growth, so they must be kept consistent across all plant groups.
- In an experiment examining the impact of sleep deprivation on cognitive performance: The time of day the cognitive tests are administered, the difficulty level of the tests, and the participants' prior knowledge would be control variables.
- In a study exploring the effectiveness of different teaching methods on student learning: The age and prior knowledge of the students, the length of the teaching sessions, and the assessment methods would be control variables.
Designing Experiments with Independent, Dependent, and Control Variables
The effective design of an experiment hinges on the careful selection and manipulation of these three variable types. A well-designed experiment allows the researcher to isolate the effect of the independent variable on the dependent variable while minimizing the influence of extraneous variables.
Formulating a Hypothesis
Before conducting an experiment, a clear hypothesis should be formulated. This hypothesis states the expected relationship between the independent and dependent variables. For example: "Increased fertilizer application will lead to increased plant growth."
Operationalizing Variables
It's crucial to define the variables operationally. This means specifying how each variable will be measured and manipulated. For example, "Fertilizer application" might be operationalized as "applying 10 grams of fertilizer per plant weekly." "Plant growth" might be operationalized as "measuring plant height in centimeters after four weeks."
Random Assignment
Random assignment of participants to different experimental groups helps ensure that extraneous variables are distributed evenly across groups. This minimizes the chances that differences in the dependent variable are due to pre-existing differences between participants rather than the manipulation of the independent variable.
Data Collection and Analysis
Once the experiment is conducted, data is collected on the dependent variable. Appropriate statistical analysis techniques are then used to determine if there is a significant relationship between the independent and dependent variables. The results of the analysis help to support or refute the initial hypothesis.
Common Mistakes to Avoid
Several common mistakes can compromise the validity and reliability of experimental research. These include:
- Confounding variables: These are variables that are not controlled and influence both the independent and dependent variables, making it difficult to determine the true effect of the independent variable.
- Lack of random assignment: Without random assignment, it’s difficult to rule out pre-existing differences between groups as explanations for observed effects.
- Poor operationalization: Vague definitions of variables make it difficult to replicate the study and interpret the results.
- Small sample size: A small sample size may not provide enough statistical power to detect a significant relationship between variables.
Advanced Considerations
As research becomes more complex, you might encounter scenarios with multiple independent variables (factorial designs) or more nuanced control strategies. Factorial designs allow investigation into the interaction effects between multiple independent variables on a single dependent variable. Sophisticated control strategies can involve statistical adjustments (e.g., analysis of covariance) to account for the influence of extraneous variables after data collection.
Conclusion: The Cornerstones of Scientific Inquiry
Understanding and effectively utilizing independent, dependent, and control variables are essential skills for anyone conducting research. By carefully defining, manipulating, and controlling these variables, researchers can confidently draw conclusions about cause-and-effect relationships and contribute meaningfully to scientific knowledge. The principles discussed in this guide are applicable across a wide range of disciplines, from psychology and biology to education and economics, ensuring the validity and reliability of your research endeavors. Mastering these concepts is a cornerstone to conducting impactful and credible research. Remember, meticulous planning, precise execution, and thorough analysis are key to unlocking valuable insights from your experimental designs.
Latest Posts
Latest Posts
-
Is Air An Element Compound Homogeneous Or Heterogeneous
Apr 08, 2025
-
Osmosis Is A Type Of Active Transport
Apr 08, 2025
-
What Is The Major Product Of This Reaction
Apr 08, 2025
-
Which Of The Following Is An Example Of Artificial Selection
Apr 08, 2025
-
How To Write A Matrix In Python
Apr 08, 2025
Related Post
Thank you for visiting our website which covers about Independent Variable Dependent Variable Control Variable . We hope the information provided has been useful to you. Feel free to contact us if you have any questions or need further assistance. See you next time and don't miss to bookmark.