How To Write A Matrix In Python
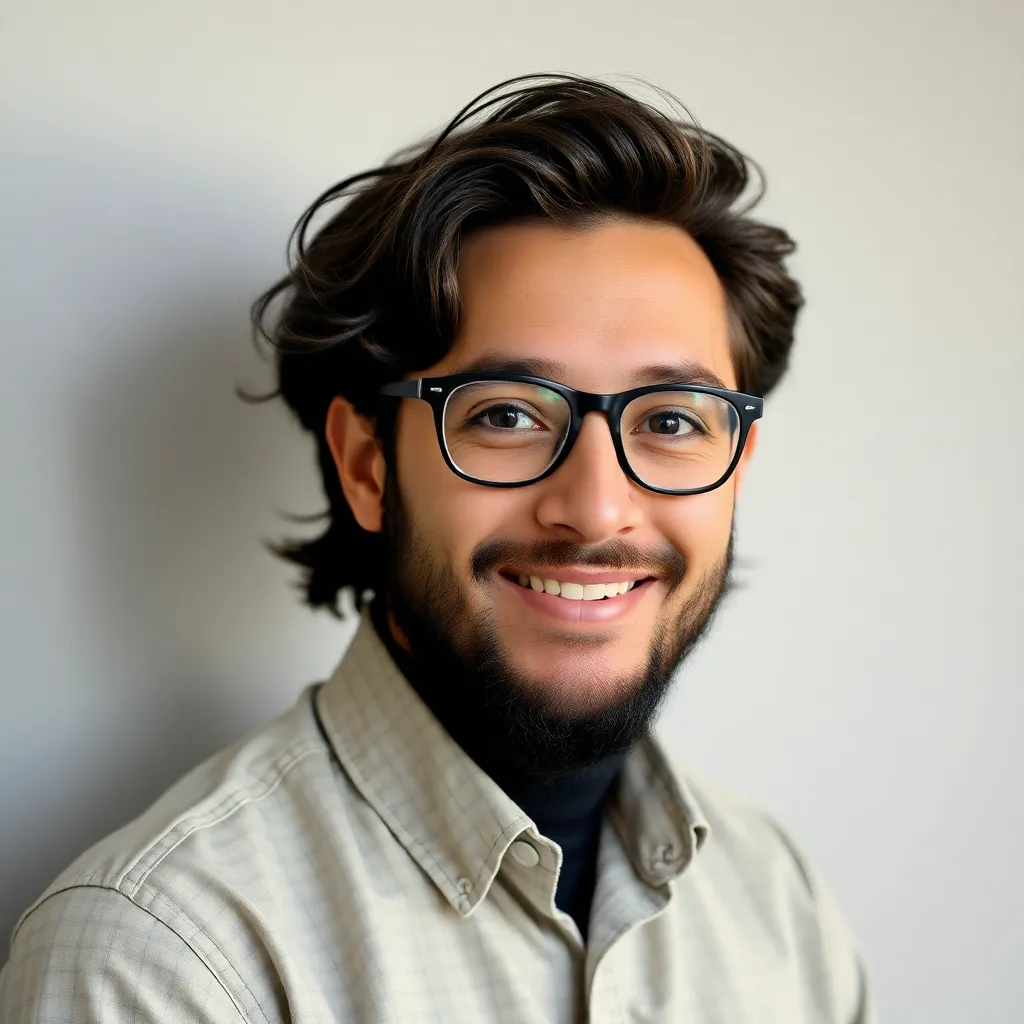
News Leon
Apr 08, 2025 · 6 min read

Table of Contents
How to Write a Matrix in Python: A Comprehensive Guide
Python, a versatile and powerful programming language, offers several ways to represent and manipulate matrices. Understanding how to effectively work with matrices is crucial for various applications, including linear algebra, data science, machine learning, and computer graphics. This comprehensive guide will explore different methods for creating and manipulating matrices in Python, covering fundamental concepts and advanced techniques.
Understanding Matrices in Python
A matrix is a two-dimensional array of numbers, symbols, or expressions arranged in rows and columns. In Python, we can represent matrices using various data structures, primarily lists of lists and NumPy arrays. Each method offers unique advantages and disadvantages depending on the specific application.
Lists of Lists: A Basic Approach
The simplest way to represent a matrix in Python is using nested lists. Each inner list represents a row of the matrix. While straightforward, this approach has limitations in terms of performance and functionalities when dealing with large matrices or complex matrix operations.
matrix_list = [
[1, 2, 3],
[4, 5, 6],
[7, 8, 9]
]
print(matrix_list)
print(matrix_list[0][1]) # Accessing element at row 0, column 1 (value: 2)
This method allows for easy creation and basic access to matrix elements. However, performing complex matrix operations (like multiplication or inversion) directly on lists of lists can be cumbersome and inefficient.
NumPy Arrays: The Efficient Choice
NumPy (Numerical Python) is a powerful library that provides optimized functionalities for numerical computations, including matrix operations. NumPy arrays are significantly more efficient than lists of lists, especially when dealing with large matrices or computationally intensive tasks. They offer optimized data structures and highly efficient functions for various matrix operations.
import numpy as np
matrix_numpy = np.array([
[1, 2, 3],
[4, 5, 6],
[7, 8, 9]
])
print(matrix_numpy)
print(matrix_numpy[0, 1]) # Accessing element at row 0, column 1 (value: 2)
print(matrix_numpy.shape) # Getting the dimensions of the matrix (3,3)
NumPy arrays provide a concise and efficient way to represent matrices and perform a wide range of operations. The np.array()
function converts a list of lists into a NumPy array. The shape
attribute provides the dimensions of the array (number of rows and columns).
Creating Matrices in Python
Let's delve into detailed methods for creating matrices using both lists of lists and NumPy arrays.
Creating Matrices Using Lists of Lists
Creating matrices using nested lists is relatively straightforward. You can manually define the elements or use loops to generate them dynamically.
# Manually creating a 3x3 identity matrix
identity_matrix = [
[1, 0, 0],
[0, 1, 0],
[0, 0, 1]
]
# Creating a 3x4 matrix with all elements initialized to 0
zero_matrix = [[0 for _ in range(4)] for _ in range(3)]
# Creating a 2x2 matrix with dynamically generated values
dynamic_matrix = [[i * j for j in range(2)] for i in range(2)]
print(identity_matrix)
print(zero_matrix)
print(dynamic_matrix)
This demonstrates creating matrices with predefined values, zero matrices, and dynamically generated matrices using nested list comprehensions for conciseness.
Creating Matrices Using NumPy
NumPy provides several functions for creating matrices efficiently. These functions offer various options for initializing matrices with specific values or patterns.
import numpy as np
# Creating a 3x3 matrix filled with zeros
zeros_np = np.zeros((3, 3))
# Creating a 2x2 matrix filled with ones
ones_np = np.ones((2, 2))
# Creating a 4x4 identity matrix
identity_np = np.identity(4)
# Creating a 3x3 matrix with random values between 0 and 1
random_np = np.random.rand(3, 3)
# Creating a 2x3 matrix with values from 1 to 6
arange_np = np.arange(1, 7).reshape(2, 3) # Reshape converts 1D array to 2D
print(zeros_np)
print(ones_np)
print(identity_np)
print(random_np)
print(arange_np)
NumPy offers functions like np.zeros()
, np.ones()
, np.identity()
, np.random.rand()
, and np.arange()
for creating matrices with different initializations and shapes. The reshape()
function allows for reshaping arrays into different dimensions.
Manipulating Matrices in Python
Once a matrix is created, various manipulations are often required. This section covers essential matrix operations using both lists of lists and NumPy arrays.
Matrix Operations with Lists of Lists
Basic operations like accessing elements, transposing, and adding/subtracting matrices can be performed, but it’s significantly less efficient than using NumPy.
matrix_a = [[1, 2], [3, 4]]
matrix_b = [[5, 6], [7, 8]]
# Accessing an element
print(matrix_a[1][0]) # Output: 3
# Adding two matrices (requires same dimensions)
matrix_sum = [[matrix_a[i][j] + matrix_b[i][j] for j in range(len(matrix_a[0]))] for i in range(len(matrix_a))]
print(matrix_sum) #Output: [[6, 8], [10, 12]]
# Transposing a matrix (swapping rows and columns) - requires manual coding
matrix_a_transpose = [[matrix_a[j][i] for j in range(len(matrix_a))] for i in range(len(matrix_a[0]))]
print(matrix_a_transpose) # Output: [[1, 3], [2, 4]]
Matrix Operations with NumPy
NumPy provides highly optimized functions for efficient matrix operations.
import numpy as np
matrix_a_np = np.array([[1, 2], [3, 4]])
matrix_b_np = np.array([[5, 6], [7, 8]])
# Addition
matrix_sum_np = matrix_a_np + matrix_b_np
print(matrix_sum_np)
# Subtraction
matrix_diff_np = matrix_a_np - matrix_b_np
print(matrix_diff_np)
# Multiplication (element-wise)
matrix_mult_elementwise_np = matrix_a_np * matrix_b_np
print(matrix_mult_elementwise_np)
# Matrix multiplication (dot product)
matrix_mult_np = np.dot(matrix_a_np, matrix_b_np)
print(matrix_mult_np)
# Transpose
matrix_transpose_np = matrix_a_np.T
print(matrix_transpose_np)
# Inverse (requires a square matrix)
matrix_inverse_np = np.linalg.inv(matrix_a_np)
print(matrix_inverse_np)
# Determinant
matrix_determinant_np = np.linalg.det(matrix_a_np)
print(matrix_determinant_np)
# Eigenvalues and Eigenvectors
eigenvalues, eigenvectors = np.linalg.eig(matrix_a_np)
print("Eigenvalues:", eigenvalues)
print("Eigenvectors:", eigenvectors)
NumPy's np.linalg
module provides a comprehensive suite of functions for linear algebra operations including addition, subtraction, element-wise multiplication, matrix multiplication (dot product), transpose, inverse, determinant, eigenvalues, and eigenvectors. These functions are significantly faster and more efficient than manual implementations using lists of lists.
Advanced Matrix Operations with NumPy
NumPy's capabilities extend beyond basic matrix operations. Let's explore more advanced functionalities:
Solving Linear Equations
NumPy's np.linalg.solve()
function can efficiently solve systems of linear equations represented in matrix form.
import numpy as np
# Coefficients matrix (A) and constants vector (b)
A = np.array([[2, 1], [1, -1]])
b = np.array([8, 1])
# Solving the linear equation Ax = b
x = np.linalg.solve(A, b)
print(x) # Output: [3. 2.]
This demonstrates how to solve a system of linear equations, a common task in various scientific and engineering applications.
Matrix Decomposition
NumPy provides functions for various matrix decompositions like LU decomposition, QR decomposition, Singular Value Decomposition (SVD), and Eigenvalue decomposition. These decompositions are fundamental in various algorithms.
import numpy as np
A = np.array([[1, 2], [3, 4]])
# LU decomposition
P, L, U = np.linalg.lu(A)
print("LU decomposition:")
print("P:", P)
print("L:", L)
print("U:", U)
# QR decomposition
Q, R = np.linalg.qr(A)
print("\nQR decomposition:")
print("Q:", Q)
print("R:", R)
# SVD
U, s, V = np.linalg.svd(A)
print("\nSVD:")
print("U:", U)
print("s:", s)
print("V:", V)
These decompositions are essential for solving linear equations, least squares problems, and other advanced computations.
Conclusion
This comprehensive guide explored various methods for creating and manipulating matrices in Python. While lists of lists offer a basic approach, NumPy arrays are strongly recommended for their efficiency and extensive functionalities. NumPy's np.linalg
module provides a powerful set of tools for performing a wide range of linear algebra operations, from simple addition and multiplication to advanced techniques like matrix decompositions and solving linear equations. Mastering these techniques is crucial for anyone working with numerical data and algorithms involving matrices in Python. Choosing the right approach – lists of lists for simple, small matrices or NumPy arrays for larger datasets and complex operations – is key to writing efficient and effective Python code. Remember to always choose the most appropriate method based on the complexity and scale of your matrix operations.
Latest Posts
Latest Posts
-
Draw The Structural Formula 2 3 3 4 Tetramethylheptane
Apr 17, 2025
-
Which Of The Following Variables Is Not Continuous
Apr 17, 2025
-
Which Of The Following Are Rational Functions Ximera
Apr 17, 2025
-
Sulphuric Acid Reaction With Sodium Hydroxide
Apr 17, 2025
-
How Many Molecules In 1 Mole Of Water
Apr 17, 2025
Related Post
Thank you for visiting our website which covers about How To Write A Matrix In Python . We hope the information provided has been useful to you. Feel free to contact us if you have any questions or need further assistance. See you next time and don't miss to bookmark.