Which Of The Following Is True Regarding The Normal Distribution
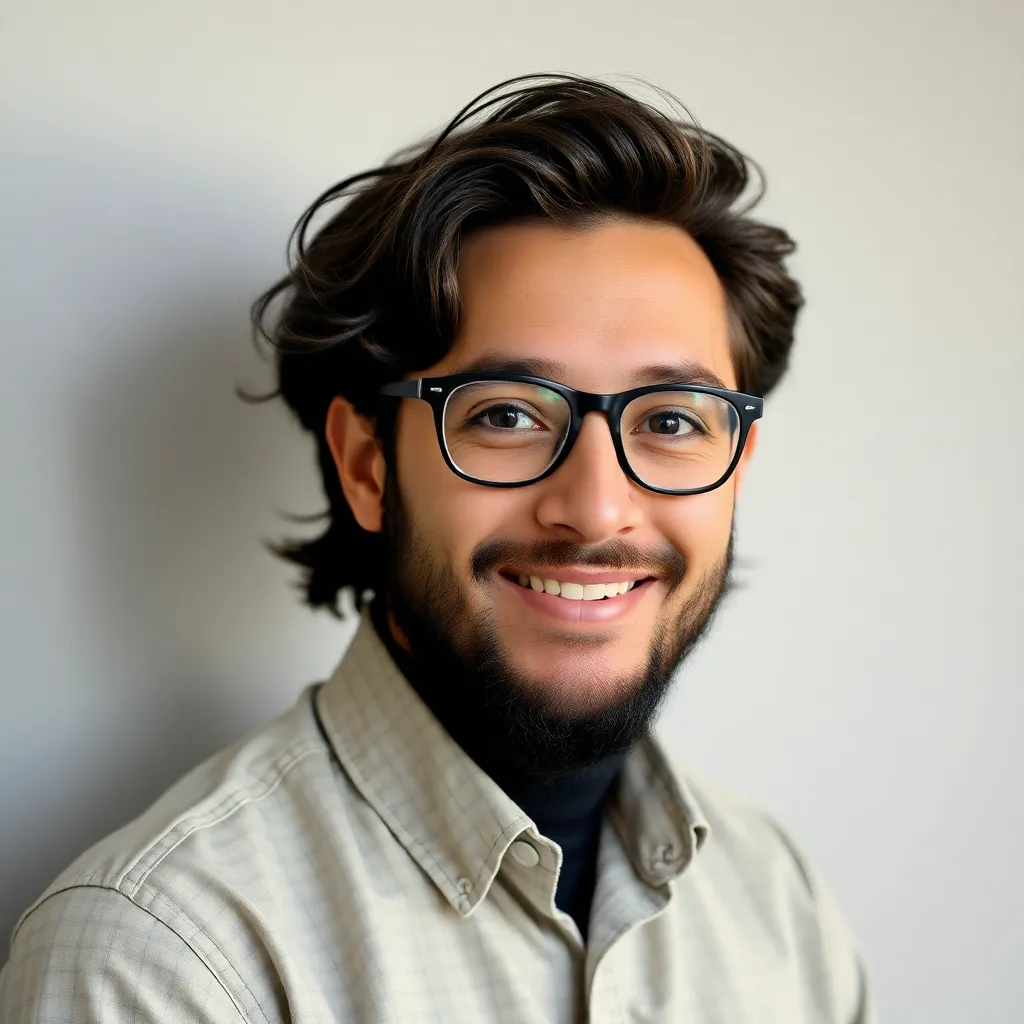
News Leon
Apr 03, 2025 · 7 min read
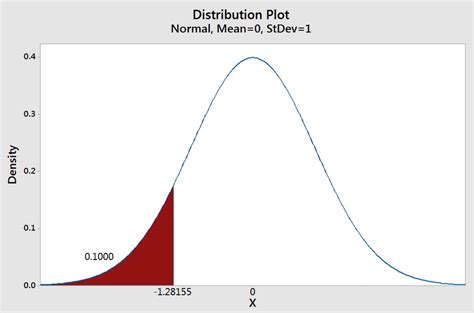
Table of Contents
Which of the Following is True Regarding the Normal Distribution?
The normal distribution, also known as the Gaussian distribution, is a cornerstone of statistics and probability. Understanding its properties is crucial for a wide range of applications, from analyzing data in scientific research to building predictive models in finance and engineering. This article delves deep into the characteristics of the normal distribution, dispelling common misconceptions and clarifying its significance. We will explore several statements regarding the normal distribution and determine their veracity. Let's tackle some common assertions:
Statement 1: The Normal Distribution is Symmetrical Around its Mean.
TRUE. This is a fundamental property of the normal distribution. Symmetry means that the distribution's shape is a mirror image on either side of the mean (µ). The mean, median, and mode are all equal in a normal distribution, and they are located at the center of the distribution. This symmetry is visually represented by the bell curve, a characteristic visual representation of the normal distribution. The probability of observing a value a certain distance below the mean is exactly equal to the probability of observing a value the same distance above the mean.
Visualizing Symmetry:
Imagine folding the bell curve in half at the mean. The two halves would perfectly overlap, demonstrating the perfect symmetry. This symmetrical nature is crucial for many statistical calculations and inferences.
Statement 2: The Normal Distribution is Defined by its Mean and Standard Deviation.
TRUE. The normal distribution is completely characterized by two parameters: the mean (µ) and the standard deviation (σ). The mean determines the location of the center of the distribution along the x-axis, while the standard deviation dictates the spread or dispersion of the data. A larger standard deviation indicates a wider, flatter distribution, whereas a smaller standard deviation indicates a narrower, taller distribution.
The Role of Mean and Standard Deviation:
- Mean (µ): Represents the average value of the distribution. It is the point of symmetry.
- Standard Deviation (σ): Measures the amount of variability or spread around the mean. Approximately 68% of the data falls within one standard deviation of the mean, 95% within two standard deviations, and 99.7% within three standard deviations (the empirical rule).
Statement 3: The Normal Distribution is Asymptotic to the x-axis.
TRUE. This means that the curve of the normal distribution gets infinitely close to the x-axis but never actually touches it. Theoretically, the tails of the distribution extend infinitely in both directions. While the probability of observing values far from the mean becomes extremely small, it's never zero. This characteristic implies that there's always a non-zero probability, albeit extremely small, of observing extreme values.
Implications of Asymptotic Nature:
The asymptotic nature of the normal distribution is important because it reflects the theoretical possibility of observing extremely high or low values, even though their probability is minuscule in practice. This is relevant in situations involving risk assessment and extreme value theory.
Statement 4: All Data Follows a Normal Distribution.
FALSE. This is a common misconception. While the normal distribution is frequently observed in many natural phenomena (e.g., height, weight, test scores), it's crucial to understand that not all data follows a normal distribution. Many datasets exhibit skewness (asymmetry), kurtosis (tailedness), or other characteristics that deviate from the symmetrical bell shape of the normal distribution.
Examples of Non-Normal Distributions:
- Income Distribution: Often highly skewed to the right, with a long tail representing high earners.
- Stock Returns: Can exhibit heavy tails, meaning that extreme values are more likely than in a normal distribution.
- Waiting Times: Often follow exponential or other non-normal distributions.
Statement 5: The Central Limit Theorem Guarantees that Sample Means Will Always Follow a Normal Distribution.
FALSE. The Central Limit Theorem (CLT) is a powerful result in statistics, stating that the sampling distribution of the mean of a large number of independent, identically distributed random variables will be approximately normally distributed, regardless of the underlying distribution of the individual variables. The crucial aspect here is "approximately" and "large number." The approximation improves as the sample size increases. For small sample sizes, especially if the underlying distribution is heavily skewed or has thick tails, the sampling distribution of the mean may not closely resemble a normal distribution.
Understanding the Central Limit Theorem:
The CLT doesn't state that individual data points follow a normal distribution, nor does it guarantee that sample means from small sample sizes will be normally distributed. It describes the behavior of the means of many samples, demonstrating that the distribution of these means tends towards normality as the sample size grows.
Statement 6: The Normal Distribution is Only Useful for Continuous Data.
FALSE. While the normal distribution is frequently used to model continuous data (e.g., height, weight, temperature), it can also be applied to discrete data in certain contexts. This often involves approximating a discrete distribution with a continuous normal distribution when the number of possible outcomes is large. The approximation improves as the number of discrete values increases. However, it’s crucial to remember this is an approximation, and its accuracy depends on the nature of the discrete data and the number of data points.
Approximating Discrete Data:
For example, if we have a large number of counts of events, we might use a normal approximation to the binomial or Poisson distribution, particularly if the conditions for such approximations are met.
Statement 7: The Standard Normal Distribution has a Mean of 0 and a Standard Deviation of 1.
TRUE. The standard normal distribution is a special case of the normal distribution where the mean (µ) is 0 and the standard deviation (σ) is 1. It's often denoted as N(0,1). Any normal distribution can be transformed into a standard normal distribution through a process called standardization or z-score transformation. This transformation involves subtracting the mean from each data point and dividing the result by the standard deviation.
Importance of Standardization:
Standardization simplifies statistical calculations and allows for comparisons between different normal distributions with varying means and standard deviations. It allows us to use standard normal tables or software to determine probabilities associated with different ranges of values.
Statement 8: The Area Under the Normal Distribution Curve Represents Probability.
TRUE. This is a fundamental concept in probability and statistics. The total area under the normal distribution curve is equal to 1, representing 100% probability. The area under a portion of the curve represents the probability that a randomly selected value from the distribution will fall within that specific range. This is why we can use the normal distribution to calculate probabilities.
Calculating Probabilities:
By using tables, software, or calculus, we can calculate the area under the curve for a specific range, providing the probability that a value will lie within that range.
Statement 9: Transforming Non-Normal Data to Normal Data is Always Necessary for Statistical Analysis.
FALSE. While the normal distribution plays a vital role in many statistical techniques, it's not always necessary to transform non-normal data to achieve normality. Many statistical methods, both parametric and non-parametric, are robust to departures from normality, especially with larger sample sizes.
When Transformation Might Be Considered:
Transformations might be considered if the data significantly violates the assumptions of a particular statistical test, but even then, non-parametric alternatives often exist. The decision of whether or not to transform data should be based on careful consideration of the specific analysis and the characteristics of the data.
Statement 10: The Normal Distribution is the Only Distribution Used in Statistics.
FALSE. While the normal distribution is incredibly important and widely used, many other distributions exist and play vital roles in statistics and probability. Different distributions are better suited to model different types of data and phenomena.
Examples of Other Important Distributions:
- Binomial Distribution: Models the probability of a certain number of successes in a fixed number of independent trials.
- Poisson Distribution: Models the probability of a certain number of events occurring in a fixed interval of time or space.
- Exponential Distribution: Models the time until an event occurs in a Poisson process.
- t-distribution: Used in hypothesis testing when the population standard deviation is unknown.
- Chi-square distribution: Used in various statistical tests, including tests of independence and goodness-of-fit.
In conclusion, understanding the properties of the normal distribution is fundamental to many statistical analyses, but it's equally important to recognize its limitations and the existence of other probability distributions. The statements examined above highlight the key features of the normal distribution and clarify some common misunderstandings about its application and limitations within the broader field of statistics. Remember, the choice of distribution to use in any given statistical analysis depends on the specific characteristics of the data and the research question being addressed.
Latest Posts
Latest Posts
-
Which Region Of The Nephron Is Impermeable To Water
Apr 04, 2025
-
Whats The Thinnest Layer Of The Earth Called
Apr 04, 2025
-
A Block Of Aluminum Occupies A Volume
Apr 04, 2025
-
Orange Yellow Pigment In Bile Formed By The Breakdown Of Hemoglobin
Apr 04, 2025
-
Which Of The Following Is An Advantage Of Globalization
Apr 04, 2025
Related Post
Thank you for visiting our website which covers about Which Of The Following Is True Regarding The Normal Distribution . We hope the information provided has been useful to you. Feel free to contact us if you have any questions or need further assistance. See you next time and don't miss to bookmark.