Which Of The Following Is Not A Misuse Of Statistics
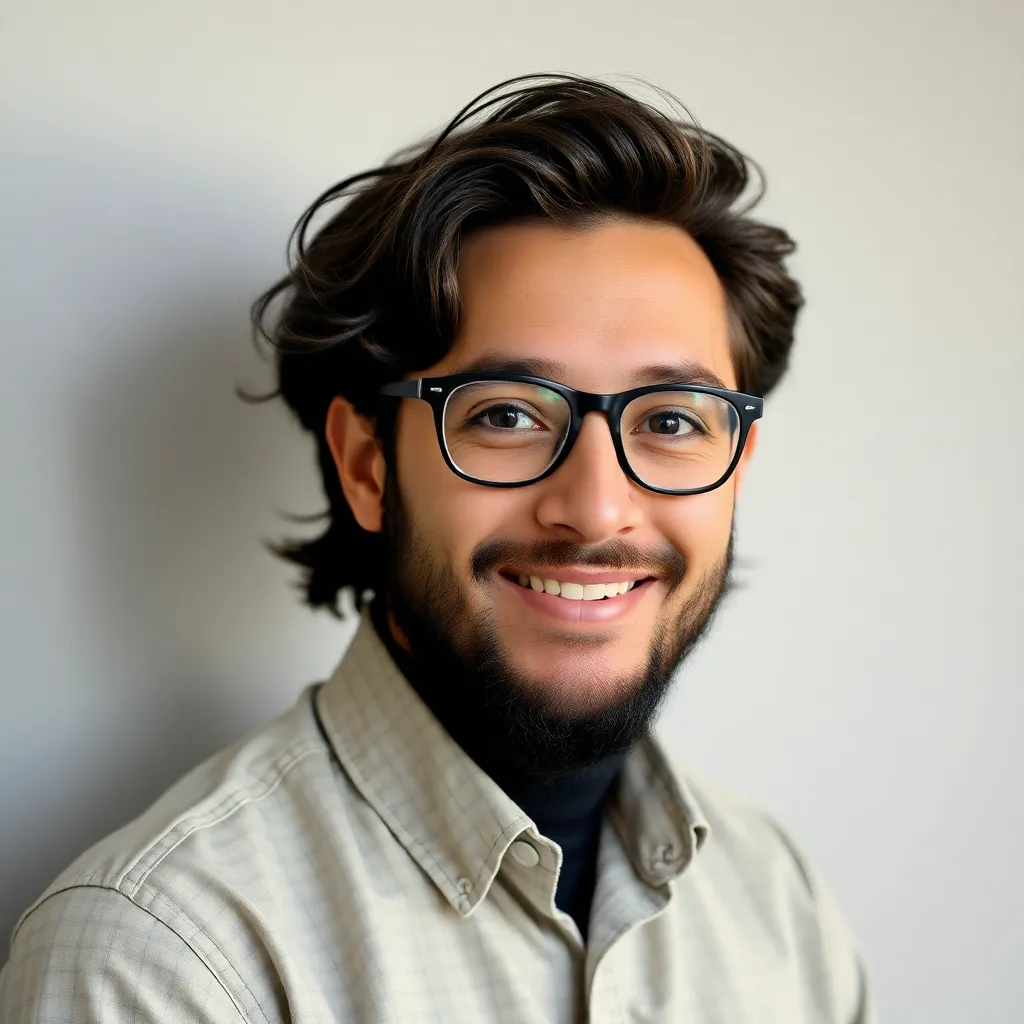
News Leon
Apr 17, 2025 · 6 min read

Table of Contents
Which of the following is NOT a Misuse of Statistics? A Deep Dive into Statistical Integrity
Statistics, a powerful tool for understanding the world around us, can be easily manipulated or misinterpreted. While often used to support claims and draw conclusions, the misuse of statistics can lead to flawed interpretations and, worse, unethical conclusions. This article delves into common misuses of statistics, highlighting how to identify them and emphasizing the importance of statistical integrity. We'll then present examples of what isn't a misuse, showcasing the responsible and ethical application of statistical analysis.
Understanding Common Misuses of Statistics
Before we can identify what isn't a misuse, we must first understand the common pitfalls:
1. Cherry-Picking Data (Selection Bias):
This involves selecting only the data points that support a pre-determined conclusion, ignoring data that contradicts it. This creates a skewed representation of the reality, leading to misleading conclusions. For instance, showcasing only positive customer reviews while ignoring negative ones.
2. Misleading Graphs and Charts:
Visual representations of data can be easily manipulated to exaggerate or downplay certain trends. Truncated y-axes, misleading scales, and improper chart types can create a false impression. Think of a bar chart where the y-axis doesn't start at zero, making small differences appear substantial.
3. Correlation vs. Causation:
Just because two variables are correlated doesn't mean one causes the other. A spurious correlation arises when a third, unmeasured variable influences both, creating a false sense of causality. For example, ice cream sales and drowning incidents are positively correlated, but one doesn't cause the other; both are influenced by the summer heat.
4. Improper Sampling Methods:
A biased sample, one not representative of the population being studied, will produce misleading results. For example, surveying only people at a high-end shopping mall about their spending habits won't reflect the spending habits of the general population.
5. Ignoring Margin of Error and Confidence Intervals:
Statistical results often come with a margin of error and a confidence interval. Ignoring these crucial elements leads to overconfident conclusions. A poll showing 55% support for a candidate with a 5% margin of error means the actual support could be anywhere between 50% and 60%.
6. Data Dredging (p-hacking):
This involves analyzing data repeatedly until a statistically significant result is found, even if it's meaningless. It increases the chance of finding false positives, which are results that appear significant by chance alone.
7. Confusing Statistical Significance with Practical Significance:
A statistically significant result doesn't automatically mean it's practically significant. A small difference might be statistically significant with a large enough sample size but might not be meaningful in the real world.
8. Insufficient Sample Size:
Using a small sample size can lead to inaccurate and unreliable conclusions. The smaller the sample, the greater the chance of results being skewed by random variation.
9. Lack of Transparency and Reproducibility:
The methodology used to collect and analyze data should be transparent and reproducible. Without this transparency, it's impossible to validate the results or assess their reliability. This is crucial for maintaining trust in the conclusions.
Examples of Responsible Statistical Practices: What IS NOT a Misuse
Now that we've examined common misuses, let's explore situations where statistics are used responsibly and ethically:
1. Presenting Complete and Accurate Data:
The most fundamental aspect of responsible statistical practice is presenting a full and accurate picture of the data. This means including all relevant data points, acknowledging limitations, and clearly presenting any caveats or uncertainties. For instance, when presenting crime statistics, including data on both reported and unreported crimes, and acknowledging the limitations of the reporting system, would constitute responsible statistical practice.
2. Using Appropriate Visualizations:
Choosing the right chart or graph is crucial for clear communication. Bar charts, line graphs, pie charts, and scatter plots each serve different purposes. Using appropriate scaling and labeling ensures the data is represented fairly and accurately. For instance, using a line graph to show trends over time, and clearly labeling the axes and units, demonstrates responsible visualization.
3. Clearly Defining Variables and Methodology:
Clearly defining the variables being studied and the methods used to collect and analyze the data is vital for reproducibility and understanding. This builds transparency and credibility. A scientific study, for example, should clearly define the independent and dependent variables, the sample size, and the statistical tests employed, allowing other researchers to replicate the study.
4. Interpreting Results in Context:
Statistical results should always be interpreted within their context. Considering external factors and potential confounding variables is crucial for drawing accurate conclusions. For example, if a study shows a correlation between exercise and weight loss, responsible interpretation would consider factors like diet, genetics, and underlying health conditions.
5. Properly Reporting Confidence Intervals and Margin of Error:
Always report confidence intervals and margins of error. These statistics provide a range of plausible values, reflecting the uncertainty inherent in statistical inference. This allows for a more nuanced understanding of the results and prevents overgeneralization.
6. Using Appropriate Statistical Tests:
Selecting the appropriate statistical test is essential for valid conclusions. Different tests are suited for different types of data and research questions. Using the wrong test can lead to incorrect interpretations. For instance, using a t-test to compare the means of two groups, or an ANOVA for comparing means of multiple groups, would be examples of using appropriate statistical tests.
7. Acknowledging Limitations and Uncertainties:
Statistical analyses are never perfect. It's crucial to acknowledge the limitations of the data and the analysis. This includes potential biases, missing data, and the inherent uncertainty associated with statistical inference. Acknowledging these limitations demonstrates intellectual honesty and promotes a more accurate understanding of the results.
8. Peer Review and Replication:
The scientific process relies on peer review and replication. Submitting work for peer review ensures that the methodology and conclusions are scrutinized by experts. Replication of studies helps to validate findings and increase confidence in the results. This process safeguards against unintentional or deliberate misrepresentations.
9. Avoiding Sensationalism and Oversimplification:
Statistical findings should be presented objectively, without sensationalizing or oversimplifying the results. Complex issues require careful explanation and should not be reduced to catchy soundbites. Responsible communication of statistical data requires a nuanced approach that accurately reflects the findings without misleading the audience.
Conclusion: The Ethical Imperative of Statistical Integrity
The responsible use of statistics is not merely a matter of technical accuracy; it's an ethical imperative. Misusing statistics can have serious consequences, leading to flawed policy decisions, distorted public understanding, and unethical manipulation. By understanding common misuses and adhering to responsible practices, we can ensure that statistics are used as a tool for truth-seeking and informed decision-making rather than a weapon for deception. A commitment to transparency, accuracy, and contextual understanding is paramount to maintaining statistical integrity and fostering trust in data-driven insights. The examples provided above offer a framework for understanding and practicing ethically sound statistical analysis, a crucial skill in our increasingly data-driven world.
Latest Posts
Latest Posts
-
Which Of The Following Is A Geometric Sequence
Apr 19, 2025
-
Why Is Aerobic Respiration More Efficient
Apr 19, 2025
-
Which Of The Following Statements Regarding Matter Is False
Apr 19, 2025
-
Is A Snake A Primary Consumer
Apr 19, 2025
-
An Economy Is Productively Efficient When
Apr 19, 2025
Related Post
Thank you for visiting our website which covers about Which Of The Following Is Not A Misuse Of Statistics . We hope the information provided has been useful to you. Feel free to contact us if you have any questions or need further assistance. See you next time and don't miss to bookmark.