Which Is Not A Measure Of Dispersion
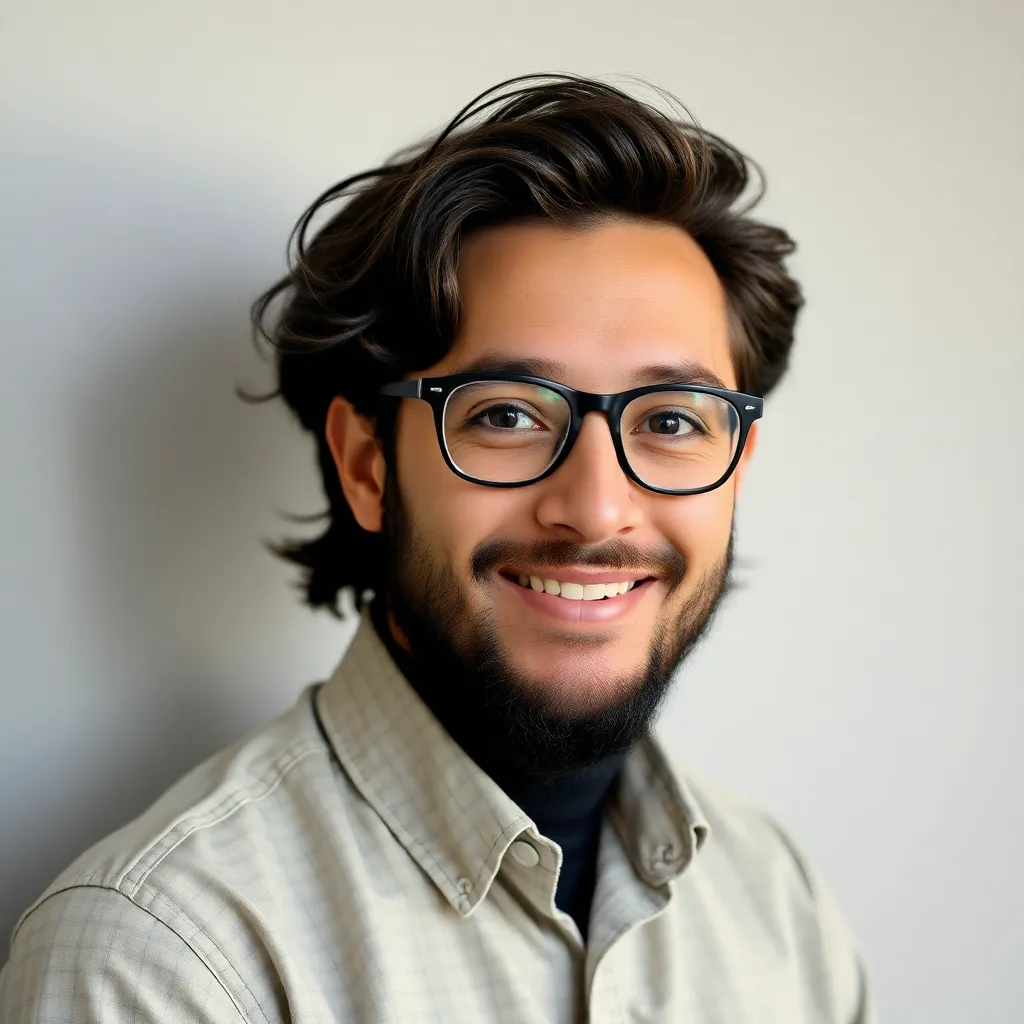
News Leon
Apr 22, 2025 · 6 min read

Table of Contents
Which is Not a Measure of Dispersion: Understanding Data Distribution
In the realm of statistics, understanding data distribution is crucial for drawing meaningful conclusions. Measures of dispersion, also known as measures of spread, quantify the variability or scatter of data points around a central tendency (like the mean or median). Knowing which statistical measures don't describe dispersion is equally important for accurate data analysis. This article delves deep into the concept of dispersion, identifying measures that are not indicators of spread and explaining why. We'll explore common statistical measures and clarify their roles in summarizing and interpreting data.
Understanding Measures of Dispersion
Before we identify what's not a measure of dispersion, let's establish a strong foundation by defining what is. Measures of dispersion describe how spread out a dataset is. A high dispersion indicates data points are far from the central value, while low dispersion means data points cluster tightly around the central value. Common measures include:
-
Range: The simplest measure, calculating the difference between the maximum and minimum values. While easy to compute, it's highly sensitive to outliers.
-
Interquartile Range (IQR): A more robust measure than the range, the IQR represents the difference between the third quartile (75th percentile) and the first quartile (25th percentile). It's less affected by extreme values.
-
Variance: The average of the squared differences from the mean. It quantifies the spread of data around the mean. A larger variance indicates greater dispersion.
-
Standard Deviation: The square root of the variance. It's expressed in the same units as the original data, making it easier to interpret than the variance. Like variance, a larger standard deviation signifies higher dispersion.
-
Mean Absolute Deviation (MAD): The average of the absolute differences from the mean. It provides another way to measure the spread around the central tendency.
Measures That Are NOT Measures of Dispersion
Several statistical measures, while important in their own right, do not describe the dispersion or spread of data. These measures focus on different aspects of the dataset:
1. Measures of Central Tendency
These measures describe the "center" or typical value of a dataset. They are fundamentally different from measures of dispersion, which describe the spread. Common measures of central tendency include:
-
Mean (Average): The sum of all values divided by the number of values. While influenced by outliers, it's a commonly used measure of central tendency.
-
Median: The middle value when the data is ordered. It's less sensitive to outliers than the mean.
-
Mode: The value that appears most frequently in the dataset. A dataset can have multiple modes or no mode at all.
Why these aren't measures of dispersion: The mean, median, and mode indicate the typical value, not how spread out the data is. For example, two datasets could have the same mean but vastly different spreads. The central tendency tells us nothing about the variability within the dataset.
2. Measures of Skewness
Skewness describes the asymmetry of a probability distribution. A symmetrical distribution has a skewness of zero. A positively skewed distribution has a longer tail on the right, while a negatively skewed distribution has a longer tail on the left. Skewness is often calculated using Pearson's moment coefficient of skewness or other similar methods.
Why skewness isn't a measure of dispersion: Skewness describes the shape of the distribution, indicating whether it's symmetrical or skewed. It doesn't directly quantify the spread or variability of the data. A highly skewed distribution can have a small or large spread, and vice-versa. A highly skewed distribution may have a small spread around the mode but a large total spread.
3. Measures of Kurtosis
Kurtosis measures the "tailedness" or "peakedness" of a distribution. It describes how heavy the tails of the distribution are compared to a normal distribution. High kurtosis indicates heavy tails and sharp peaks (leptokurtic), while low kurtosis indicates light tails and flat peaks (platykurtic).
Why kurtosis isn't a measure of dispersion: Kurtosis focuses on the shape of the distribution's tails and peak, not the spread of the data. Two distributions could have the same kurtosis but very different dispersions. A high kurtosis might imply a concentration of data near the mean, making the spread seem low despite the tails.
4. Probability and Percentiles
While probabilities and percentiles (like quartiles) are related to data distribution, they don't directly measure dispersion.
-
Probabilities: These describe the likelihood of an event occurring. They don't inherently describe the spread of data.
-
Percentiles: These divide the data into hundredths. While used in calculating the IQR (which is a measure of dispersion), percentiles themselves don't quantify the spread. For example, knowing the 25th and 75th percentiles is useful but doesn't fully describe the dispersion without calculating the IQR.
Why probabilities and percentiles aren't measures of dispersion (on their own): They provide information about the cumulative distribution function (CDF) but not a direct measure of the data's spread or variability.
5. Coefficient of Variation (CV) – A nuanced case
The coefficient of variation (CV) is the ratio of the standard deviation to the mean, often expressed as a percentage. While it uses the standard deviation (a measure of dispersion), the CV itself is not a direct measure of dispersion. It's a relative measure of dispersion, showing the variability relative to the mean.
Why CV is not a direct measure of dispersion: A high CV indicates high variability relative to the mean, but the absolute spread might still be small if the mean is also small. Similarly, a low CV might suggest low variability, but the absolute spread might be large if the mean is large. The CV is useful for comparing the variability of datasets with different units or scales, not for measuring the absolute dispersion.
Distinguishing Between Measures: A Practical Example
Let's consider two datasets to illustrate the difference between measures of central tendency and measures of dispersion:
Dataset A: {10, 10, 10, 10, 10} Dataset B: {1, 5, 10, 15, 19}
Both datasets have a mean of 10. However, the dispersion is drastically different.
-
Dataset A: Has a range of 0, an IQR of 0, variance of 0, standard deviation of 0, and MAD of 0. This indicates no dispersion – all data points are identical.
-
Dataset B: Has a range of 18, a larger IQR, a significant variance and standard deviation, and a non-zero MAD. This shows considerable dispersion.
This example clearly shows that measures of central tendency (like the mean) alone are insufficient to describe data. Measures of dispersion are necessary to fully understand data variability.
Conclusion: Choosing the Right Measure
Selecting the appropriate statistical measure depends heavily on the nature of the data and the research question. While measures of central tendency provide insight into the typical value, understanding data spread requires measures of dispersion. Failing to consider dispersion can lead to inaccurate conclusions and a flawed understanding of data. Therefore, it is crucial to carefully consider the characteristics of the data and select the appropriate descriptive statistics, including those that quantify dispersion accurately. Remember that the mean, median, mode, skewness, kurtosis, probabilities, and percentiles, while crucial statistical tools, do not, on their own, serve as measures of the data's spread or variability. Choosing the right combination of measures is critical for drawing informed conclusions from your data analysis.
Latest Posts
Latest Posts
-
A Pure Monopoly May Generate Economic Profits Because
Apr 23, 2025
-
A Block Of Mass 2 0 Kg Starting From Rest
Apr 23, 2025
-
How Many Electrons Can 3d Hold
Apr 23, 2025
-
Is Orange Juice With Pulp Homogeneous Or Heterogeneous
Apr 23, 2025
-
Are Seismic Waves Mechanical Or Electromagnetic Waves
Apr 23, 2025
Related Post
Thank you for visiting our website which covers about Which Is Not A Measure Of Dispersion . We hope the information provided has been useful to you. Feel free to contact us if you have any questions or need further assistance. See you next time and don't miss to bookmark.