What Numbers Cannot Be A Probability
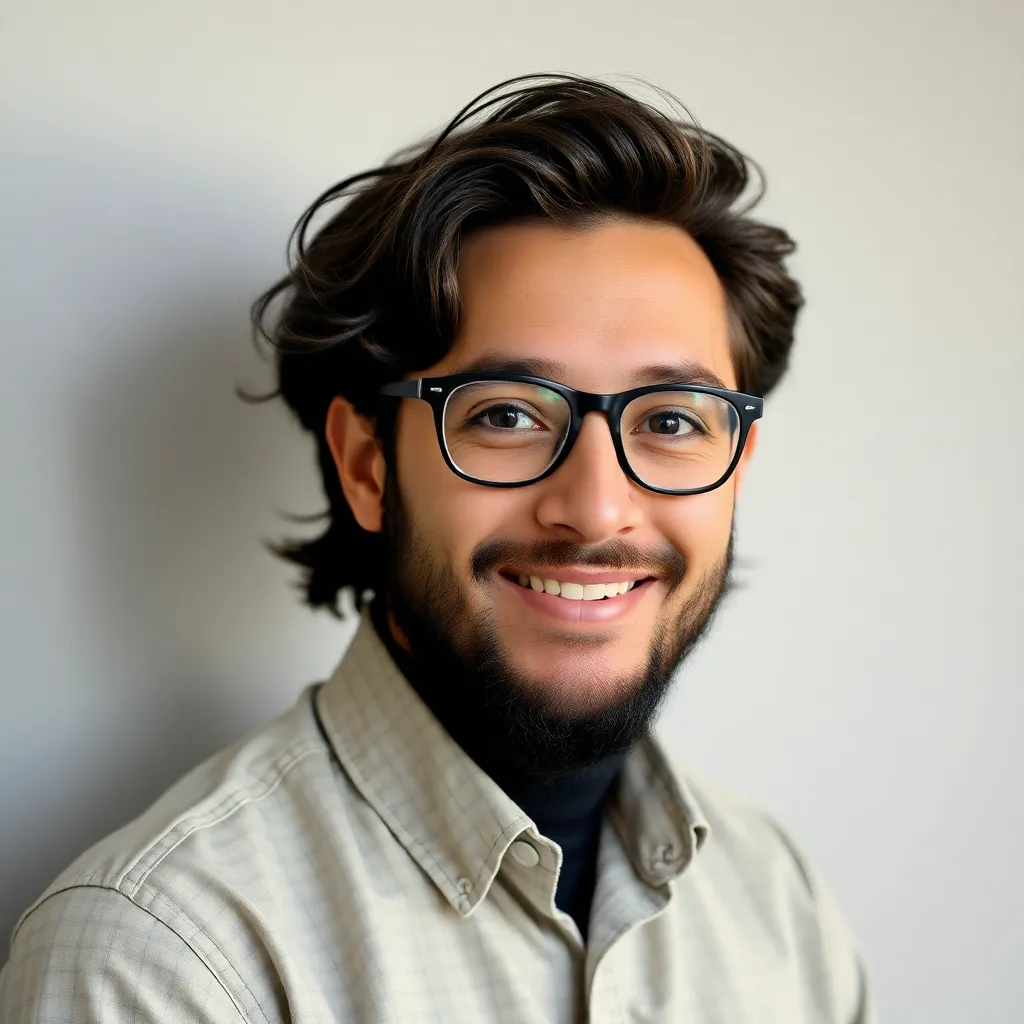
News Leon
Apr 06, 2025 · 6 min read
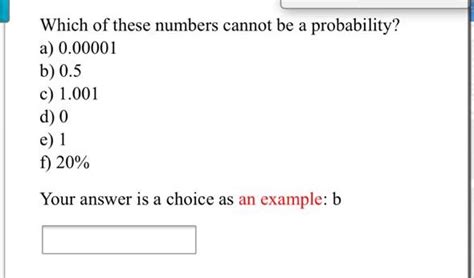
Table of Contents
What Numbers Cannot Be a Probability?
Probability, at its core, quantifies the likelihood of an event occurring. It's a fundamental concept in statistics, mathematics, and countless real-world applications, from predicting weather patterns to assessing financial risk. Understanding what numbers cannot represent a probability is just as crucial as understanding what numbers can. This article delves deep into the constraints that define valid probability values, exploring the mathematical underpinnings and illustrating the concepts with practical examples.
The Fundamental Rule: Probability Lies Between 0 and 1
The most fundamental rule governing probabilities is that they must always fall within the range of 0 to 1, inclusive. This is often represented mathematically as:
0 ≤ P(A) ≤ 1
Where:
-
P(A) represents the probability of event A occurring.
-
0 signifies impossibility – the event absolutely will not happen.
-
1 signifies certainty – the event is guaranteed to happen.
Any number outside this range is simply not a valid probability. Let's examine why this is so crucial:
Why Probabilities Cannot Be Less Than 0
A probability less than 0 is nonsensical because it implies a level of impossibility that exceeds the absence of the event. Probability quantifies the likelihood of occurrence; a negative probability suggests a "negative likelihood," a concept without meaning in the context of probability theory. It's like saying the chances of rain are -20%; this is not a valid description of the possibility of rain.
Why Probabilities Cannot Be Greater Than 1
Similarly, a probability greater than 1 suggests a likelihood exceeding certainty. If the probability of an event is 1.2, for instance, it indicates a certainty plus an additional 0.2 certainty. This is contradictory to the very definition of probability, as an event cannot be more certain than certain. Imagine claiming the probability of the sun rising tomorrow is 1.5 – this statement is illogical and violates the foundational principles of probability.
Practical Examples of Invalid Probabilities
Let's consider some scenarios to illustrate the concept of invalid probabilities:
Scenario 1: Predicting the Outcome of a Coin Toss
The probability of getting heads in a fair coin toss is 0.5 (or 50%). This is a valid probability because it falls within the 0-1 range. However, if someone claims the probability is 1.5, it's immediately clear this is incorrect. You cannot have a higher chance than 100% of getting heads. Similarly, claiming the probability is -0.2 is equally wrong; you can't have a negative probability of obtaining heads.
Scenario 2: Weather Forecasting
A weather forecaster might predict a 70% chance of rain. This is a valid probability (0.7). But if they predict a 120% chance of rain, it demonstrates a misunderstanding of probabilities. The maximum chance of rain is 100%, representing absolute certainty. Similarly, a negative probability of rain is nonsensical.
Scenario 3: Medical Diagnosis
A doctor might assess the probability of a patient having a specific disease as 0.8 (80%). This is acceptable, reflecting a high likelihood but not absolute certainty. However, a probability of 1.3 is not valid. The patient cannot have a 130% chance of having the disease.
Beyond the Basic Range: The Importance of Completeness
While the 0-1 range is paramount, it's also crucial to consider the completeness of the probability assignment for all possible outcomes within an event space. The sum of probabilities for all mutually exclusive and collectively exhaustive outcomes must equal 1. This is because one of the possible outcomes must occur.
Mutually Exclusive Events
Mutually exclusive events are events that cannot happen simultaneously. For example, in a single coin toss, you cannot get both heads and tails.
Collectively Exhaustive Events
Collectively exhaustive events encompass all possible outcomes in a given scenario. In the coin toss example, heads and tails are collectively exhaustive because there are no other possibilities.
Example: If we assign a probability of 0.6 to the event "it will rain tomorrow" and a probability of 0.3 to the event "it will not rain tomorrow," we have a problem. The probabilities add up to 0.9, not 1. This means there's a missing 0.1 probability. The probability space is not complete; we've failed to account for all possible outcomes. To resolve this, we need to reconsider the probability assignments to ensure they add up to 1.
Probability Distributions and Their Constraints
Probability distributions provide a comprehensive framework for describing the probabilities of different outcomes in various scenarios. Different types of probability distributions impose their own constraints.
For example, a normal distribution is defined over the entire real number line. However, while a normal distribution can take on values across the whole spectrum, the probability associated with each part of the distribution must be between 0 and 1. The integral of the probability density function over its entire range must equal 1. This means that if you were to calculate the probability of a certain range of the distribution, the answer would lie between 0 and 1.
Similarly, a Poisson distribution, used to model the probability of a given number of events occurring in a fixed interval, has probabilities for each possible non-negative integer number of occurrences. Each individual probability within the Poisson distribution must remain within the 0-1 range, and the sum of probabilities for all possible occurrences must equal 1.
Misinterpretations and Common Mistakes
Several common misinterpretations lead to incorrect probability assignments:
-
Confusing probability with frequency: Probability is a theoretical measure of likelihood, while frequency is an observed count of occurrences in a sample. A high frequency in a sample does not necessarily mean a high probability, especially with small sample sizes.
-
Ignoring conditional probabilities: The probability of an event often depends on other events. Ignoring these dependencies can lead to incorrect calculations. For example, the probability of rain may be different given that it is already cloudy.
-
Overlooking the sample space: Failing to properly define the sample space—all possible outcomes—can lead to incomplete probability assignments and erroneous calculations.
Conclusion
Understanding what numbers cannot be a probability is fundamental to working with probability and statistics correctly. The 0-1 constraint, the need for complete probability spaces, and the considerations imposed by various probability distributions are all critical aspects of this understanding. By adhering to these rules and avoiding common pitfalls, we can ensure accurate and meaningful interpretations of probability in diverse applications. Incorrect probability assignments not only lead to flawed conclusions but can have serious implications in fields ranging from risk management to medical diagnosis. Therefore, a thorough grasp of the permissible range and constraints of probability is essential for anyone working with this crucial concept.
Latest Posts
Latest Posts
-
Contain Carbon Hydrogen Oxygen And Nitrogen
Apr 09, 2025
-
What Is The Empirical Formula For Ibuprofen
Apr 09, 2025
Related Post
Thank you for visiting our website which covers about What Numbers Cannot Be A Probability . We hope the information provided has been useful to you. Feel free to contact us if you have any questions or need further assistance. See you next time and don't miss to bookmark.