What Inference Can Be Drawn From The Graph
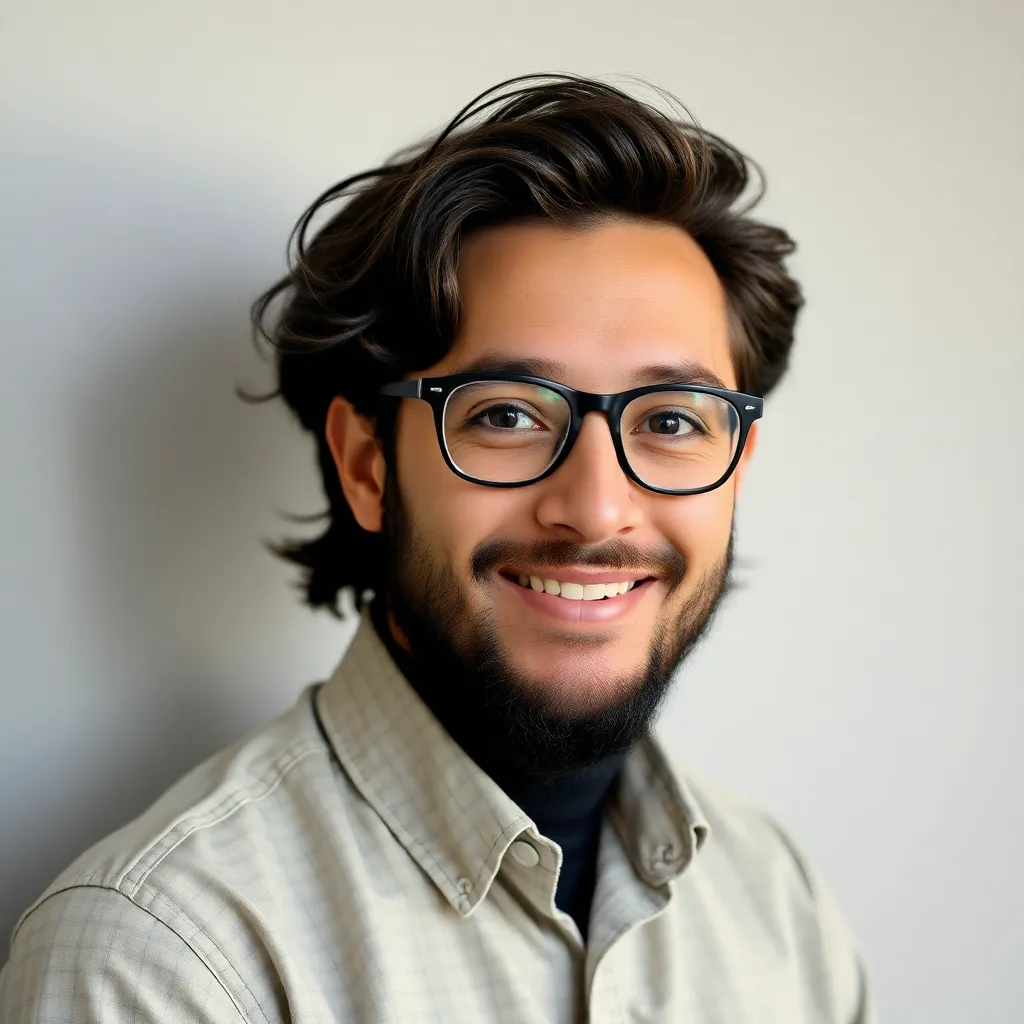
News Leon
Apr 21, 2025 · 6 min read

Table of Contents
What Inferences Can Be Drawn From the Graph? A Comprehensive Guide
Graphs are powerful tools for visualizing data and communicating insights. They condense complex information into easily digestible formats, allowing for quick identification of trends, patterns, and anomalies. However, simply looking at a graph isn't enough; extracting meaningful inferences requires careful analysis and understanding of the context. This article will delve into the process of drawing inferences from graphs, covering various graph types and the nuances of interpretation.
Understanding the Basics: Types of Graphs and Their Uses
Before we dive into inference, let's review some common graph types and their typical applications:
1. Line Graphs: Showing Trends Over Time
Line graphs excel at illustrating changes in a variable over time. They are ideal for demonstrating growth, decline, or cyclical patterns. Key inferences often drawn from line graphs include:
- Trends: Is there an overall upward or downward trend? Is the trend linear or exponential? Are there periods of rapid growth or decline?
- Turning Points: Are there points where the trend changes direction (e.g., peaks, troughs, inflection points)? What factors might have contributed to these changes?
- Seasonality: Does the data exhibit seasonal fluctuations? If so, what is the pattern and amplitude of these fluctuations?
- Comparisons: If multiple lines are plotted, how do they compare in terms of trends and magnitudes? Are there periods of convergence or divergence?
Example: A line graph showing monthly sales of a product over a year could reveal peak sales during the holiday season and a general upward trend indicating market growth. The inference might be that holiday marketing campaigns are effective and that the product is gaining popularity.
2. Bar Graphs: Comparing Categories
Bar graphs are effective for comparing discrete categories. They visually represent the magnitude of a variable for each category. Inferences often drawn from bar graphs include:
- Magnitude Comparisons: Which category has the highest/lowest value? How do the values compare across categories?
- Proportions: What proportion of the total does each category represent? Are there any significantly disproportionate categories?
- Rankings: What is the ranking of categories from highest to lowest? Are there significant differences between rankings?
- Groupings: Can categories be grouped based on similar values? Are there clusters of categories with similar characteristics?
Example: A bar graph showing the sales of different product lines in a company could reveal which product line is most profitable and which ones are underperforming. The inference might be a need to reallocate resources to boost sales of underperforming lines.
3. Pie Charts: Showing Proportions
Pie charts are excellent for representing proportions or percentages of a whole. They are particularly useful when illustrating the relative contribution of different parts to a total. Inferences often drawn from pie charts include:
- Dominant Categories: Which category represents the largest proportion of the whole? Are there any significantly small categories?
- Relative Sizes: How do the proportions of different categories compare to each other? Are there any unexpected proportions?
- Changes Over Time: If multiple pie charts are compared (e.g., for different time periods), how have the proportions changed?
Example: A pie chart showing the distribution of customer demographics (age, gender, location) could reveal which demographic segment represents the largest customer base. The inference might be to tailor marketing efforts towards that dominant segment.
4. Scatter Plots: Showing Correlations
Scatter plots are used to visualize the relationship between two continuous variables. They reveal correlations – positive, negative, or no correlation – between the variables. Inferences drawn from scatter plots include:
- Correlation Strength: Is there a strong, moderate, or weak correlation between the variables? Is the relationship positive (as one variable increases, so does the other), negative (as one variable increases, the other decreases), or non-existent?
- Outliers: Are there any data points that deviate significantly from the overall pattern? These outliers might warrant further investigation.
- Linearity: Is the relationship between the variables approximately linear or non-linear? This helps determine the type of model that might best describe the relationship.
- Causation vs. Correlation: It is crucial to remember that correlation does not imply causation. A scatter plot can show a strong correlation, but it doesn't necessarily mean that one variable causes the change in the other.
Example: A scatter plot showing the relationship between advertising expenditure and sales could reveal a positive correlation, suggesting that increased advertising leads to increased sales. However, it's important to consider other factors that might be contributing to the sales increase.
The Process of Drawing Inferences: A Step-by-Step Approach
Drawing accurate inferences requires a systematic approach:
-
Understand the Context: Before analyzing the graph, understand the data it represents. What variables are being measured? What is the time period or population being considered? What are the units of measurement?
-
Identify Key Features: Look for the most prominent features of the graph – trends, peaks, troughs, outliers, correlations, and proportions.
-
Interpret the Data: What do the key features suggest? What patterns or relationships are evident?
-
Consider External Factors: Are there any external factors that might influence the data? These factors could include economic conditions, seasonal variations, or policy changes.
-
Formulate Inferences: Based on your analysis and consideration of external factors, formulate logical inferences. These inferences should be supported by evidence from the graph.
-
Evaluate the Limitations: Acknowledge any limitations of the data or the graph itself. For instance, the data might be limited to a specific region or time period, or the graph might not accurately represent complex relationships.
-
Communicate Your Findings: Clearly and concisely communicate your inferences, supporting them with specific details from the graph.
Advanced Techniques for Inference
For more complex graphs or datasets, advanced statistical techniques may be needed to extract meaningful inferences. These can include:
- Regression Analysis: This technique is used to model the relationship between variables and predict future values.
- Time Series Analysis: This technique is used to analyze data collected over time, identifying trends, seasonality, and other patterns.
- Clustering Analysis: This technique is used to group similar data points together, revealing underlying structures in the data.
- Hypothesis Testing: This technique is used to test whether observed differences in data are statistically significant.
Avoiding Common Pitfalls in Inference
Several common mistakes can lead to inaccurate inferences from graphs:
- Ignoring Context: Failing to consider the context of the data can lead to misinterpretations.
- Oversimplification: Assuming a simple linear relationship when a more complex relationship exists.
- Causation vs. Correlation: Mistaking correlation for causation.
- Ignoring Outliers: Failing to investigate outliers that might reveal important information.
- Misleading Scales: Graphs with manipulated scales can distort the data and lead to incorrect inferences.
Conclusion: The Power of Informed Inference
Graphs are valuable tools for data visualization and communication, but extracting meaningful inferences requires more than just a cursory glance. By following a systematic approach, understanding the different types of graphs, and avoiding common pitfalls, you can unlock the power of data visualization and draw valuable insights from your graphs. The ability to accurately interpret and communicate these insights is crucial for informed decision-making in a wide range of fields. Remember, the goal is not just to see the data but to understand what it means and what actions might be warranted based on that understanding. Through careful consideration and critical analysis, graphs can become powerful tools for revealing the hidden stories within data.
Latest Posts
Latest Posts
-
Choose The Correct Statement Concerning Electric Field Lines
Apr 21, 2025
-
Thirty Five Thousandths In Decimal Form
Apr 21, 2025
-
A Negative Ion Is Known As A
Apr 21, 2025
-
What Is The Formula For Lithium Fluoride
Apr 21, 2025
-
Blood Flow Is Slow Through Capillaries Because Capillaries
Apr 21, 2025
Related Post
Thank you for visiting our website which covers about What Inference Can Be Drawn From The Graph . We hope the information provided has been useful to you. Feel free to contact us if you have any questions or need further assistance. See you next time and don't miss to bookmark.