The Square Root Of The Variance Is Called The
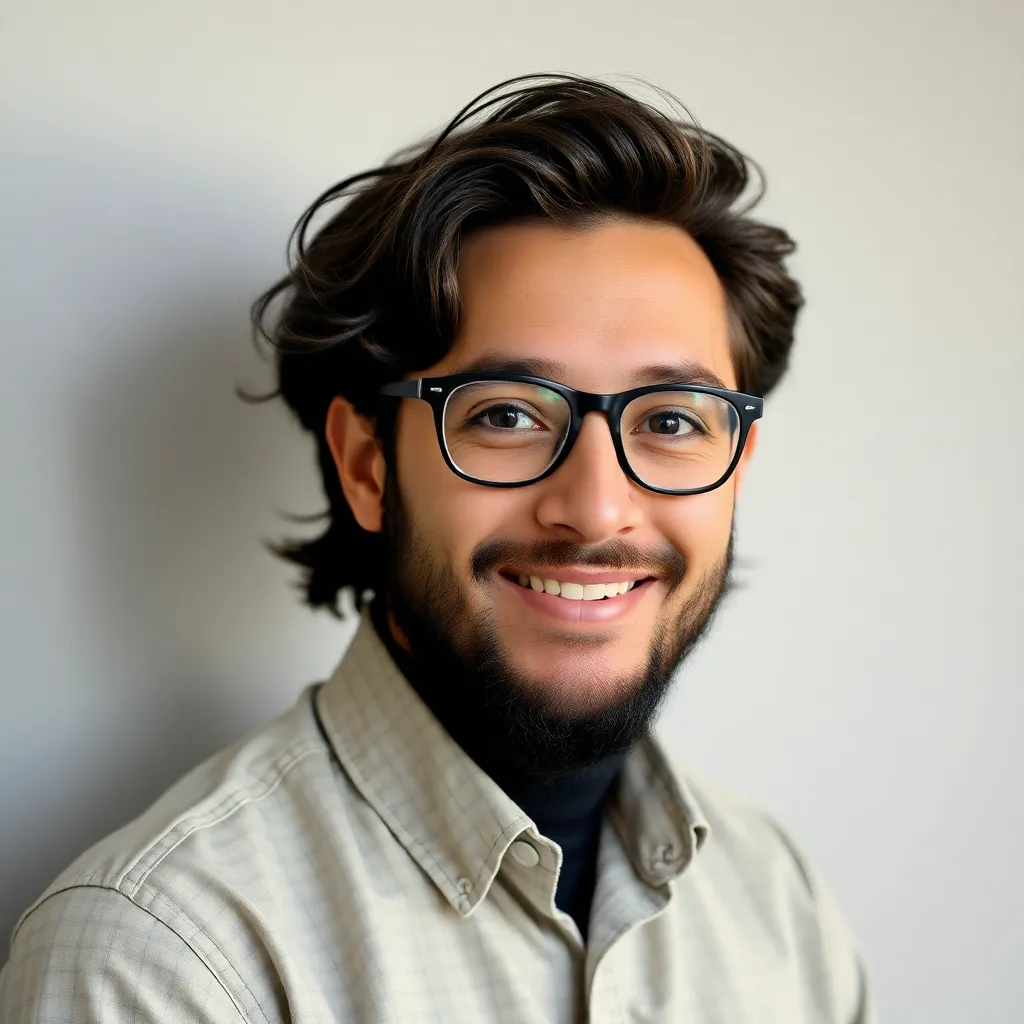
News Leon
Apr 16, 2025 · 6 min read

Table of Contents
The Square Root of the Variance is Called the Standard Deviation: A Deep Dive
The square root of the variance is called the standard deviation. This seemingly simple statement underpins a vast amount of statistical analysis, data interpretation, and decision-making across numerous fields. Understanding standard deviation goes beyond simply knowing the definition; it requires grasping its implications, calculations, applications, and interpretations within different contexts. This comprehensive guide will explore all these aspects, equipping you with a thorough understanding of this fundamental statistical concept.
Understanding Variance and its Limitations
Before delving into the standard deviation, it's crucial to understand its precursor: variance. Variance measures the average squared deviation of each data point from the mean. In simpler terms, it quantifies the spread or dispersion of data around the central tendency. A high variance indicates a wide spread of data points, while a low variance indicates data points clustered closely around the mean.
The formula for variance (σ²) in a population is:
σ² = Σ(xᵢ - μ)² / N
Where:
- σ² represents the population variance.
- xᵢ represents each individual data point.
- μ represents the population mean.
- N represents the total number of data points in the population.
For a sample, the formula slightly changes to account for the sample's tendency to underestimate population variance:
s² = Σ(xᵢ - x̄)² / (n - 1)
Where:
- s² represents the sample variance.
- x̄ represents the sample mean.
- n represents the total number of data points in the sample.
While variance provides a valuable measure of dispersion, it has a significant drawback: its units are squared. This means that the units of variance are not directly comparable to the original data's units. For example, if your data represents heights in centimeters, the variance will be in square centimeters, a less intuitive unit for interpretation. This is where the standard deviation steps in.
Standard Deviation: The Square Root Solution
The standard deviation (σ or s) resolves the issue of squared units by simply taking the square root of the variance. This returns a measure of dispersion in the original units of the data, making it significantly more interpretable and directly comparable to the data itself. Therefore, the standard deviation represents the average distance of each data point from the mean.
For a population: σ = √σ² = √[Σ(xᵢ - μ)² / N]
For a sample: s = √s² = √[Σ(xᵢ - x̄)² / (n - 1)]
Interpreting Standard Deviation
The magnitude of the standard deviation offers crucial insights into data distribution:
- Small Standard Deviation: Indicates that the data points are clustered tightly around the mean, suggesting low variability.
- Large Standard Deviation: Indicates that the data points are spread widely around the mean, suggesting high variability.
Applications of Standard Deviation Across Disciplines
The standard deviation's applications are widespread, spanning numerous fields:
1. Finance: Risk Assessment
In finance, standard deviation is a critical measure of investment risk. A higher standard deviation for an investment indicates greater volatility and, consequently, higher risk. Investors use this metric to compare the risk profiles of different investment options.
2. Quality Control: Process Variability
In manufacturing and quality control, the standard deviation helps assess the consistency of a production process. A low standard deviation signifies a stable and reliable process, producing products with consistent quality. Conversely, a high standard deviation indicates inconsistent product quality, necessitating process improvements.
3. Healthcare: Clinical Trials
Clinical trials extensively utilize standard deviation to analyze the variability in treatment responses. This helps determine the effectiveness and safety of new drugs or therapies. A smaller standard deviation in treatment outcomes suggests a more consistent and predictable response.
4. Meteorology: Weather Forecasting
Meteorologists use standard deviation to analyze weather patterns and predict future conditions. For example, the standard deviation of daily temperatures over a period can provide insights into the temperature variability expected in a particular region.
5. Education: Student Performance Analysis
In education, standard deviation helps analyze student performance on tests or assessments. It quantifies the spread of scores, aiding in identifying areas where students are performing consistently well or poorly. This information can be crucial for tailoring teaching strategies and providing targeted support.
Calculating Standard Deviation: A Step-by-Step Guide
Let's illustrate the calculation of standard deviation with a practical example:
Consider the following dataset representing the daily sales of a small business over a week:
- Monday: $100
- Tuesday: $150
- Wednesday: $120
- Thursday: $180
- Friday: $110
Step 1: Calculate the mean (x̄):
x̄ = (100 + 150 + 120 + 180 + 110) / 5 = $132
Step 2: Calculate the deviations from the mean (xᵢ - x̄):
- Monday: 100 - 132 = -32
- Tuesday: 150 - 132 = 18
- Wednesday: 120 - 132 = -12
- Thursday: 180 - 132 = 48
- Friday: 110 - 132 = -22
Step 3: Square the deviations:
- Monday: (-32)² = 1024
- Tuesday: (18)² = 324
- Wednesday: (-12)² = 144
- Thursday: (48)² = 2304
- Friday: (-22)² = 484
Step 4: Sum the squared deviations:
Σ(xᵢ - x̄)² = 1024 + 324 + 144 + 2304 + 484 = 4280
Step 5: Divide by (n - 1) to get the sample variance (s²):
s² = 4280 / (5 - 1) = 1070
Step 6: Take the square root to obtain the sample standard deviation (s):
s = √1070 ≈ $32.71
Therefore, the sample standard deviation of daily sales is approximately $32.71. This indicates that the daily sales fluctuate, on average, by about $32.71 around the mean daily sales of $132.
Standard Deviation and Normal Distribution
The standard deviation plays a particularly important role when dealing with normally distributed data. The normal distribution, often depicted as a bell curve, is a symmetrical probability distribution where data is concentrated around the mean. In a normal distribution:
- Approximately 68% of the data falls within one standard deviation of the mean.
- Approximately 95% of the data falls within two standard deviations of the mean.
- Approximately 99.7% of the data falls within three standard deviations of the mean.
This characteristic of the normal distribution makes the standard deviation invaluable for understanding the probability of observing specific data points within a dataset.
Standard Deviation vs. Other Measures of Dispersion
While standard deviation is a widely used measure of dispersion, it's essential to understand its relationship with other measures, such as:
- Range: The difference between the maximum and minimum values in a dataset. The range is simple to calculate but highly sensitive to outliers.
- Interquartile Range (IQR): The difference between the 75th and 25th percentiles. The IQR is less sensitive to outliers than the range.
- Mean Absolute Deviation (MAD): The average of the absolute deviations from the mean. MAD is less sensitive to outliers than standard deviation but less commonly used.
The choice of dispersion measure depends on the specific dataset and the research question. Standard deviation is often preferred when dealing with normally distributed data, while other measures may be more suitable for skewed or outlier-prone datasets.
Conclusion: The Importance of Understanding Standard Deviation
The square root of the variance, the standard deviation, is a cornerstone of statistical analysis. Its ability to provide a readily interpretable measure of data dispersion in the original units makes it invaluable across a broad spectrum of applications. Understanding its calculation, interpretation, and implications within different contexts is crucial for anyone working with data, regardless of their field. From assessing investment risk to ensuring product quality, the standard deviation offers a powerful tool for understanding and interpreting data, driving informed decision-making and advancing knowledge across numerous disciplines. By grasping the fundamental concepts outlined in this article, you'll be well-equipped to harness the power of standard deviation in your own analyses.
Latest Posts
Latest Posts
-
What State Is Chlorine At Room Temperature
Apr 19, 2025
-
Benzaldehyde And Acetone Aldol Condensation Mechanism
Apr 19, 2025
-
Give One Example Of A Chemical Change
Apr 19, 2025
-
A Compound A Has The Formula C8h10
Apr 19, 2025
-
Is Milk Of Magnesia An Acid Or A Base
Apr 19, 2025
Related Post
Thank you for visiting our website which covers about The Square Root Of The Variance Is Called The . We hope the information provided has been useful to you. Feel free to contact us if you have any questions or need further assistance. See you next time and don't miss to bookmark.