Standard Deviation And Coefficient Of Variance
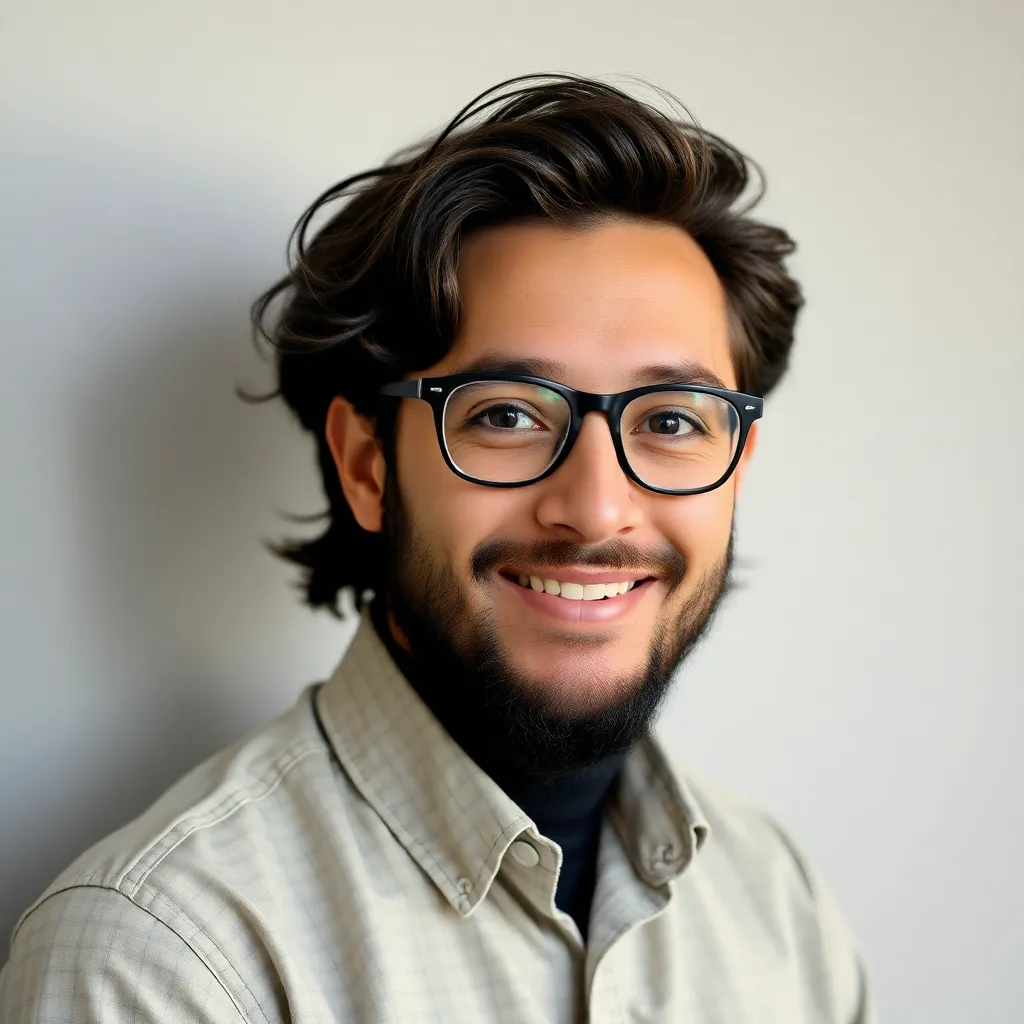
News Leon
Apr 17, 2025 · 6 min read

Table of Contents
Understanding Standard Deviation and Coefficient of Variance: A Comprehensive Guide
Standard deviation and coefficient of variance are crucial statistical measures used to describe the dispersion or spread of a dataset. While both quantify variability, they do so in different ways, making them suitable for different applications. This comprehensive guide will delve into the meaning, calculation, interpretation, and applications of both standard deviation and the coefficient of variance, equipping you with a robust understanding of these essential statistical concepts.
What is Standard Deviation?
Standard deviation measures the average distance of each data point from the mean (average) of the dataset. A higher standard deviation indicates greater variability, meaning the data points are more spread out, while a lower standard deviation signifies less variability, with data points clustered closer to the mean. Think of it as a measure of how much the individual data points "deviate" from the typical value.
Calculating Standard Deviation: A Step-by-Step Guide
The calculation of standard deviation involves several steps:
-
Calculate the mean (average): Sum all the data points and divide by the number of data points.
-
Calculate the deviations: Subtract the mean from each data point. These are your individual deviations.
-
Square the deviations: Square each of the deviations obtained in step 2. This removes negative signs and emphasizes larger deviations.
-
Calculate the variance: Sum the squared deviations and divide by the number of data points minus 1 (for sample standard deviation) or by the number of data points (for population standard deviation). Variance is the average of the squared deviations. Using 'n-1' for sample standard deviation provides an unbiased estimate of the population variance.
-
Calculate the standard deviation: Take the square root of the variance. This gives you the standard deviation, expressed in the same units as the original data.
Example:
Let's say we have the following dataset: 2, 4, 4, 6, 8.
-
Mean: (2 + 4 + 4 + 6 + 8) / 5 = 4.8
-
Deviations: 2 - 4.8 = -2.8; 4 - 4.8 = -0.8; 4 - 4.8 = -0.8; 6 - 4.8 = 1.2; 8 - 4.8 = 3.2
-
Squared Deviations: (-2.8)² = 7.84; (-0.8)² = 0.64; (-0.8)² = 0.64; (1.2)² = 1.44; (3.2)² = 10.24
-
Variance (sample): (7.84 + 0.64 + 0.64 + 1.44 + 10.24) / (5 - 1) = 5.2
-
Standard Deviation (sample): √5.2 ≈ 2.28
Interpreting Standard Deviation
A larger standard deviation indicates greater variability or spread in the data, while a smaller standard deviation suggests the data points are clustered tightly around the mean. It's essential to consider the context of the data and the units of measurement when interpreting the standard deviation. For instance, a standard deviation of 2.28 in the example above might be considered large or small depending on the context of the data being measured.
What is Coefficient of Variance (CV)?
The coefficient of variance (CV), also known as relative standard deviation, is a normalized measure of dispersion. Unlike the standard deviation, which is expressed in the same units as the data, the CV is a dimensionless quantity, expressed as a percentage. This makes it particularly useful for comparing the variability of datasets with different units or scales.
Calculating Coefficient of Variance
The coefficient of variance is calculated by dividing the standard deviation by the mean and multiplying by 100% to express it as a percentage:
CV = (Standard Deviation / Mean) * 100%
Example:
Using the same dataset from the standard deviation example (2, 4, 4, 6, 8):
- Standard Deviation: ≈ 2.28
- Mean: 4.8
- CV: (2.28 / 4.8) * 100% ≈ 47.5%
Interpreting Coefficient of Variance
The CV provides a relative measure of variability, allowing for comparison across different datasets. A higher CV indicates greater relative variability compared to the mean, while a lower CV suggests less relative variability. For example, a CV of 47.5% suggests a relatively high degree of variability in the example dataset compared to its mean. However, this needs to be considered in context with other datasets.
Standard Deviation vs. Coefficient of Variance: Key Differences and Applications
While both standard deviation and coefficient of variance measure variability, they serve different purposes:
Feature | Standard Deviation | Coefficient of Variance |
---|---|---|
Units | Same units as the data | Dimensionless (percentage) |
Interpretation | Absolute measure of dispersion | Relative measure of dispersion |
Comparison | Difficult to compare across different datasets | Easy to compare across different datasets |
Applications | Describing spread of data within a single dataset | Comparing variability across datasets with different units or scales |
When to use Standard Deviation:
- When you need an absolute measure of dispersion within a single dataset.
- When the units of measurement are important for interpretation.
- When comparing datasets with similar means and scales.
When to use Coefficient of Variance:
- When comparing the variability of datasets with different units or scales.
- When the magnitude of the mean is significantly different across datasets.
- When you need a standardized measure of dispersion that is independent of the units of measurement.
Advanced Applications and Considerations
Both standard deviation and coefficient of variance find extensive applications across various fields:
- Finance: Assessing risk in investment portfolios, comparing volatility of different asset classes.
- Quality Control: Monitoring process variability, identifying sources of variation in manufacturing processes.
- Healthcare: Evaluating the variability of patient outcomes, assessing the effectiveness of treatments.
- Environmental Science: Analyzing variability in environmental data, comparing pollution levels across different regions.
- Engineering: Evaluating the reliability of components, assessing the variability of product performance.
Considerations:
- Outliers: Both standard deviation and CV are sensitive to outliers. Extreme values can significantly influence the calculated values. Robust statistical methods might be needed for datasets with outliers.
- Data Distribution: Standard deviation is most meaningfully interpreted for data that is approximately normally distributed. For highly skewed distributions, other measures of dispersion may be more appropriate.
- Sample Size: The accuracy of the standard deviation and CV estimates increases with sample size.
Conclusion
Standard deviation and coefficient of variance are fundamental statistical tools for analyzing and interpreting data variability. Understanding their differences and applications is crucial for drawing meaningful conclusions from data analysis. While standard deviation provides an absolute measure of dispersion, the coefficient of variance offers a normalized measure for comparing variability across datasets with different scales. Choosing the appropriate measure depends on the specific research question and the characteristics of the data being analyzed. By mastering these concepts, researchers and analysts can gain deeper insights from their data and make more informed decisions. Remember to always consider the context of your data and the potential impact of outliers when interpreting these statistical measures. This comprehensive understanding will empower you to effectively communicate your findings and contribute significantly to your field of study.
Latest Posts
Latest Posts
-
Which Material Cannot Be Made Into A Magnet
Apr 19, 2025
-
What Is The Net Gain Of Atp From Glycolysis
Apr 19, 2025
-
Gravitational Force Between The Sun And The Earth
Apr 19, 2025
-
What Is The Ph Of A Neutral Substance
Apr 19, 2025
-
Why Cation Is Smaller Than Its Parent Atom
Apr 19, 2025
Related Post
Thank you for visiting our website which covers about Standard Deviation And Coefficient Of Variance . We hope the information provided has been useful to you. Feel free to contact us if you have any questions or need further assistance. See you next time and don't miss to bookmark.