How Many Independent Variables Can You Have In An Experiment
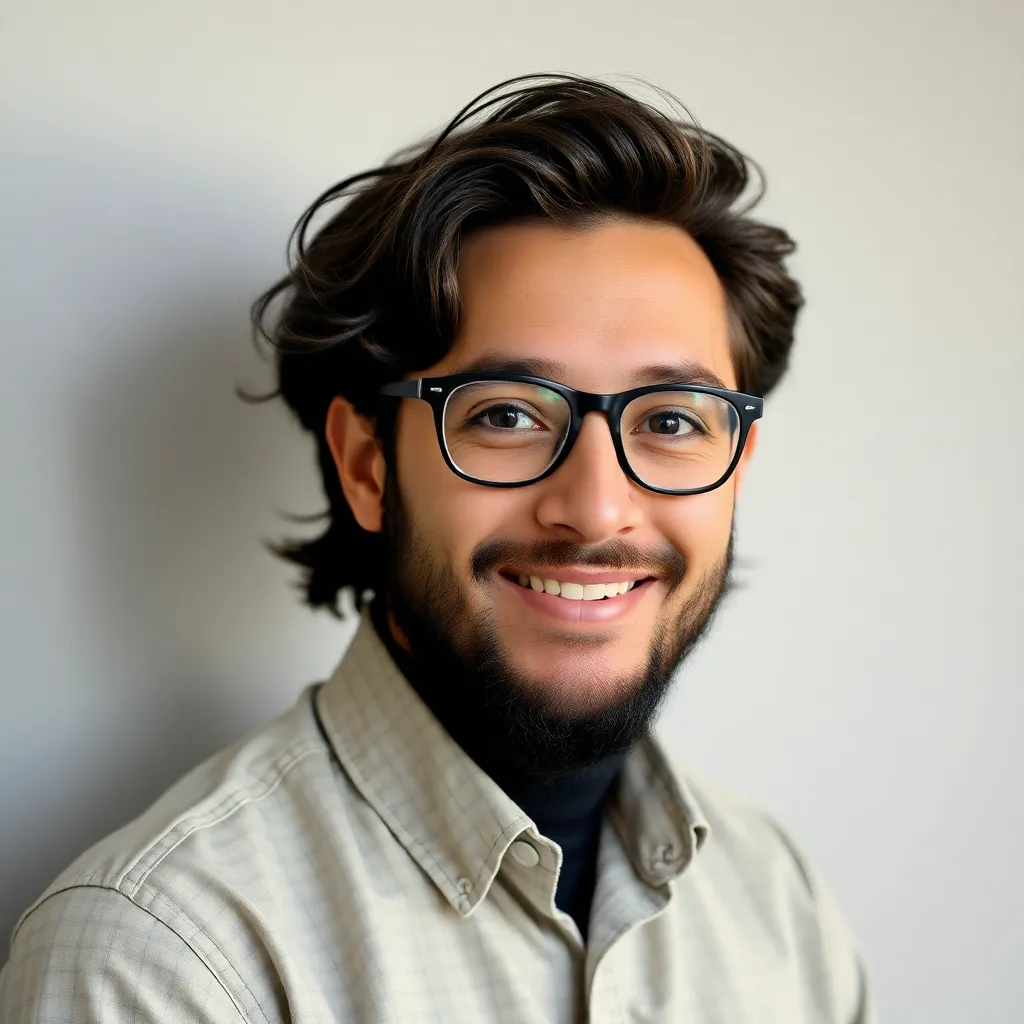
News Leon
Apr 15, 2025 · 6 min read
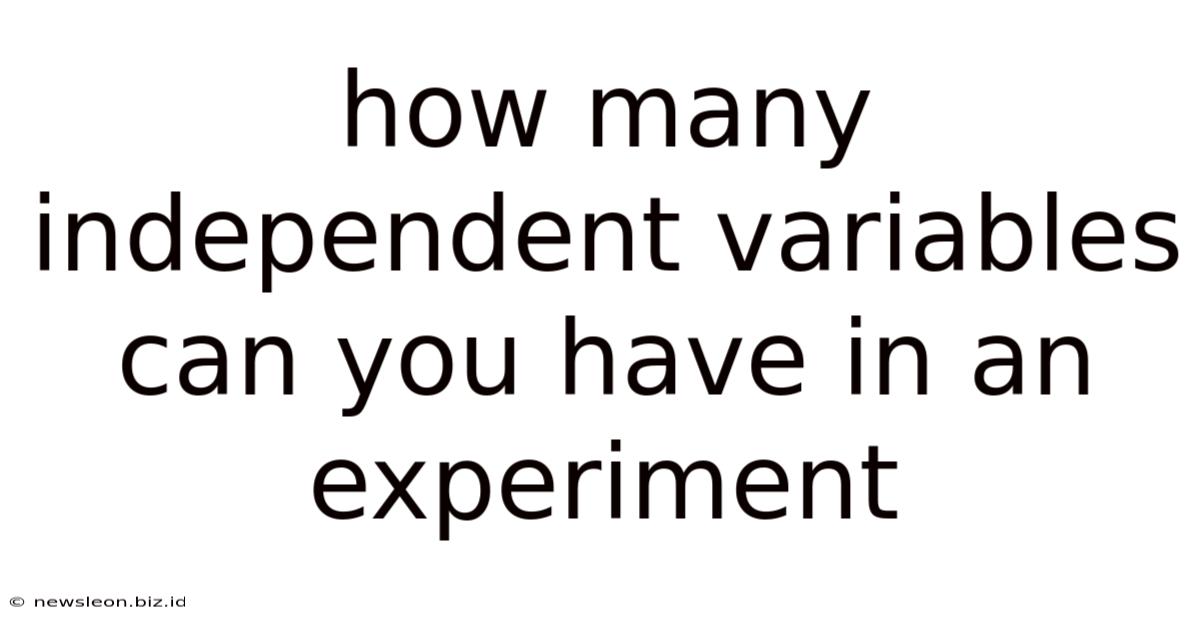
Table of Contents
How Many Independent Variables Can You Have in an Experiment?
Determining the optimal number of independent variables in your experiment is a crucial decision impacting the validity, reliability, and feasibility of your research. There's no single, universally correct answer; the ideal number depends on several interacting factors, including your research question, resources, and the complexity of the phenomenon under investigation. This article delves deep into the considerations involved in choosing the right number of independent variables, exploring the advantages and disadvantages of using multiple independent variables and providing practical guidelines to aid your decision-making process.
Understanding Independent Variables
Before diving into the number of independent variables, let's establish a clear understanding of what they are. An independent variable is the variable that is manipulated or changed by the researcher to observe its effect on the dependent variable. It's the presumed cause in a cause-and-effect relationship. For instance, in an experiment testing the effect of different fertilizers on plant growth, the type of fertilizer is the independent variable, while plant growth is the dependent variable.
The Advantages of Multiple Independent Variables
Employing multiple independent variables in a single experiment offers several significant advantages:
1. Investigating Complex Interactions:
Many real-world phenomena are not influenced by a single factor but by the interplay of multiple variables. Using multiple independent variables allows researchers to explore these interactions, revealing how different independent variables influence each other's effect on the dependent variable. For example, studying the impact of both fertilizer type and watering frequency on plant growth allows you to determine if the effect of fertilizer depends on the watering schedule, and vice-versa. This provides a more nuanced and realistic understanding of the phenomenon.
2. Increased Efficiency:
Conducting multiple experiments, each focusing on a single independent variable, can be time-consuming and resource-intensive. A single experiment with multiple independent variables can be far more efficient, allowing researchers to gather more data and achieve greater breadth of knowledge in less time and with fewer resources.
3. Enhanced Generalizability:
Experiments with multiple independent variables can lead to findings with higher generalizability. By examining the impact of several factors simultaneously, you can better understand how the results apply to more complex real-world scenarios where multiple factors are at play. This is particularly important when aiming to translate research findings into practical applications.
The Disadvantages of Multiple Independent Variables
While using multiple independent variables offers advantages, it also comes with potential drawbacks:
1. Increased Complexity:
As the number of independent variables increases, the experimental design becomes significantly more complex. This complexity can lead to difficulties in:
- Designing the experiment: Creating a well-controlled experiment with numerous variables requires careful planning and meticulous attention to detail.
- Interpreting the results: Analyzing the data and interpreting the results become significantly more challenging, particularly when dealing with complex interactions between variables.
- Controlling extraneous variables: With more variables, the risk of extraneous variables confounding the results increases. This necessitates more rigorous control measures.
2. Increased Resource Demands:
Experiments with multiple independent variables generally require more participants, more materials, and more time to conduct. This can lead to increased costs and logistical challenges, making such experiments less feasible for researchers with limited resources.
3. Higher Risk of Type I Error:
With multiple independent variables and multiple statistical tests, the risk of committing a Type I error (false positive) increases. This is the risk of concluding that there is a significant effect when there is actually no effect. Careful statistical planning and adjustment of alpha levels (significance levels) are crucial to mitigate this risk.
4. Diminishing Returns:
Beyond a certain point, adding more independent variables may yield diminishing returns. The additional information gained may not be proportional to the increased complexity and resources required.
Determining the Optimal Number: Practical Guidelines
The optimal number of independent variables depends on a variety of factors. There's no magic number, but consider these guidelines:
-
Research Question: The complexity of your research question dictates the number of independent variables needed. A simple question may only require one or two, while a complex question may necessitate more. Clearly define your research question before determining the number of independent variables.
-
Resources: Your available resources (time, budget, participants, materials) constrain the number of variables you can realistically handle. Be realistic about your resource limitations and design an experiment that is feasible within those constraints.
-
Experimental Design: Different experimental designs (e.g., factorial design, between-subjects design, within-subjects design) have different capacities for handling multiple independent variables. Choose a design appropriate for the number of variables you intend to use.
-
Statistical Power: The statistical power of your experiment—the probability of detecting a real effect if one exists—is influenced by the number of variables and participants. Ensure sufficient statistical power to detect meaningful effects.
-
Prior Research: Review existing literature to see how similar studies have addressed the relationship between your variables. This can guide your decision and help you avoid unnecessary variables.
Examples and Scenarios
Let's consider a few scenarios to illustrate the interplay of these factors:
Scenario 1: Simple Experiment
Research Question: Does caffeine intake affect reaction time?
Independent Variable: Caffeine dosage (low, medium, high).
This simple question requires only one independent variable.
Scenario 2: Moderately Complex Experiment
Research Question: How do different teaching methods and student motivation levels affect academic performance?
Independent Variables: Teaching method (lecture, group work, individual study) and student motivation level (high, low).
This requires two independent variables, allowing for investigation of their individual effects and their interaction.
Scenario 3: Complex Experiment
Research Question: What is the impact of different fertilizer types, watering frequencies, and sunlight exposure on the yield and quality of tomatoes?
Independent Variables: Fertilizer type (A, B, C), Watering frequency (daily, every other day), Sunlight exposure (full sun, partial shade).
This is a more complex experiment requiring three independent variables, leading to a factorial design with many experimental conditions. Careful planning and resource allocation are vital.
Conclusion
Determining the appropriate number of independent variables in an experiment is a balancing act. While multiple independent variables can provide richer insights and greater efficiency, they increase complexity, resource demands, and the risk of statistical errors. By carefully considering your research question, available resources, experimental design, and statistical power, you can make an informed decision about the optimal number of independent variables for your study, ensuring a rigorous, manageable, and insightful research endeavor. Remember, prioritizing clarity and feasibility is just as important as ambition in experimental design. Start with a well-defined research question and progressively add independent variables only when necessary and feasible.
Latest Posts
Related Post
Thank you for visiting our website which covers about How Many Independent Variables Can You Have In An Experiment . We hope the information provided has been useful to you. Feel free to contact us if you have any questions or need further assistance. See you next time and don't miss to bookmark.