Find The Variance Of The Probability Distribution
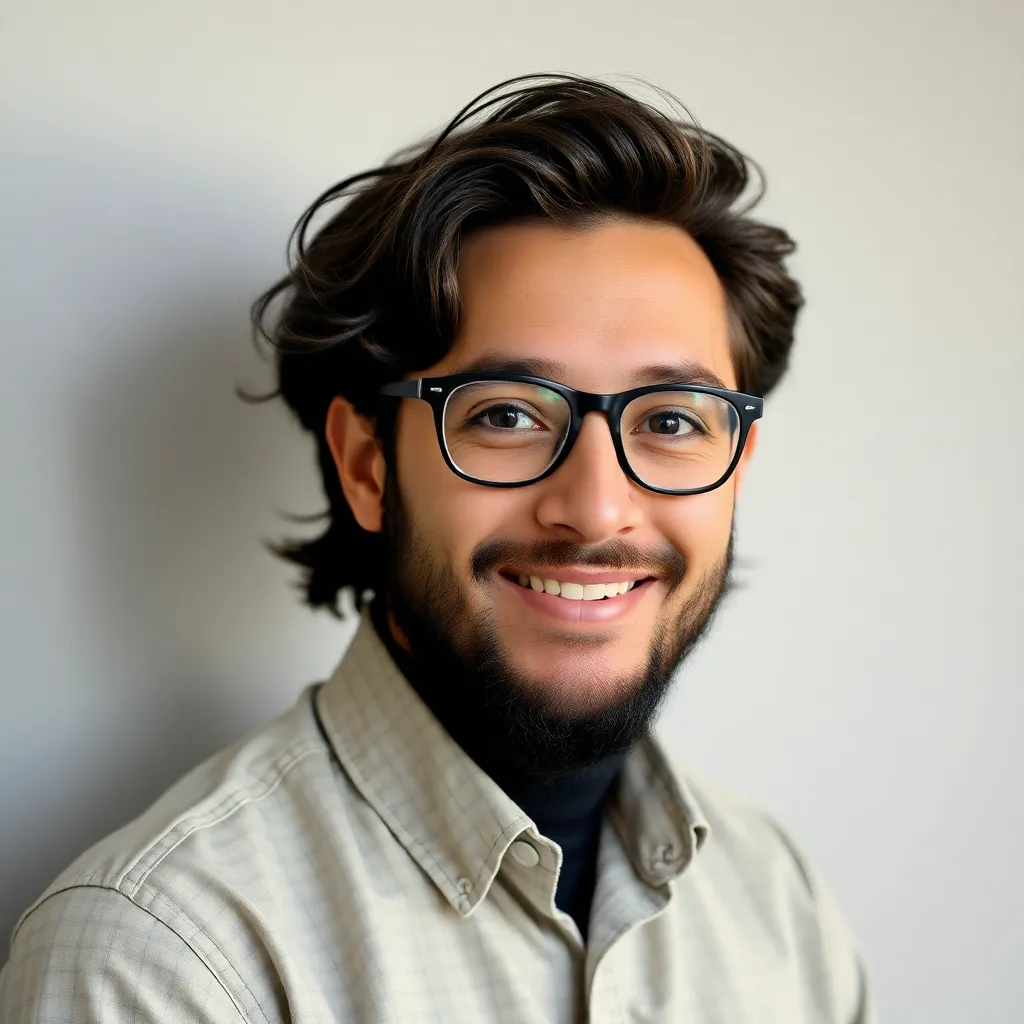
News Leon
Apr 27, 2025 · 5 min read

Table of Contents
Finding the Variance of a Probability Distribution: A Comprehensive Guide
Understanding the variance of a probability distribution is crucial in statistics. It measures how spread out the distribution is, indicating the dispersion of data points around the mean. A high variance suggests data points are far from the mean, while a low variance indicates data points cluster closely around the mean. This guide will comprehensively explain how to find the variance, covering various probability distributions and providing practical examples.
What is Variance?
Variance (σ²) is a statistical measure quantifying the spread or dispersion of a set of data points. It represents the average of the squared differences from the mean. A larger variance implies greater variability, meaning the data points are more scattered. Conversely, a smaller variance signifies less variability, indicating data points are clustered closer to the mean. Understanding variance helps us grasp the uncertainty or risk associated with a particular variable.
Why is Variance Important?
- Risk Assessment: In finance, variance is used to measure the risk associated with an investment. A higher variance indicates higher risk.
- Data Analysis: It helps in understanding the spread and distribution of data, allowing for more informed decisions.
- Model Comparison: Comparing the variances of different models helps determine which model best fits the data.
- Process Control: In quality control, variance helps monitor the consistency of a process.
Calculating Variance for Different Probability Distributions
The method for calculating variance differs slightly depending on the type of probability distribution. We will explore the most common ones:
1. Discrete Probability Distribution
For a discrete probability distribution, the variance is calculated using the following formula:
σ² = Σ [(xᵢ - μ)² * P(xᵢ)]
Where:
- xᵢ: Represents each value of the random variable.
- μ: Represents the mean (expected value) of the distribution.
- P(xᵢ): Represents the probability of each value xᵢ.
- Σ: Represents the summation over all possible values of xᵢ.
Example:
Let's consider a discrete random variable X with the following probability distribution:
X | P(X) |
---|---|
1 | 0.2 |
2 | 0.3 |
3 | 0.5 |
1. Calculate the mean (μ):
μ = (1 * 0.2) + (2 * 0.3) + (3 * 0.5) = 2.3
2. Calculate the variance (σ²):
σ² = [(1 - 2.3)² * 0.2] + [(2 - 2.3)² * 0.3] + [(3 - 2.3)² * 0.5] = 0.51
2. Continuous Probability Distribution
For a continuous probability distribution, the variance is calculated using the following formula:
σ² = ∫ (x - μ)² f(x) dx
Where:
- x: Represents the random variable.
- μ: Represents the mean of the distribution.
- f(x): Represents the probability density function.
- ∫: Represents the integral over the entire range of x.
This calculation often requires calculus. Let's look at examples for specific continuous distributions:
a) Normal Distribution
The variance of a normal distribution with mean μ and standard deviation σ is simply σ². The standard deviation (σ) is the square root of the variance. Therefore, if you know the standard deviation, you automatically know the variance.
b) Exponential Distribution
The exponential distribution with parameter λ has a mean of 1/λ and a variance of 1/λ².
c) Uniform Distribution
A uniform distribution on the interval [a, b] has a mean of (a+b)/2 and a variance of (b-a)²/12.
3. Using the Shortcut Formula for Variance
Calculating variance directly using the basic formula can be tedious, especially with large datasets. A shortcut formula exists that simplifies the calculation:
σ² = E(X²) - [E(X)]²
Where:
- E(X): Represents the expected value (mean) of X.
- E(X²): Represents the expected value of X².
This formula is applicable to both discrete and continuous distributions. First, you calculate E(X) and E(X²). Then, substitute these values into the formula to obtain the variance.
Example (using shortcut formula for discrete distribution):
Using the same discrete distribution from the previous example:
1. Calculate E(X): (As before) E(X) = μ = 2.3
2. Calculate E(X²):
E(X²) = (1² * 0.2) + (2² * 0.3) + (3² * 0.5) = 5.9
3. Calculate the variance:
σ² = E(X²) - [E(X)]² = 5.9 - (2.3)² = 0.51 (Same result as before!)
Understanding Standard Deviation
The standard deviation (σ) is the square root of the variance (σ²). It's often preferred over variance because it's expressed in the same units as the original data, making it easier to interpret. The standard deviation provides a measure of the typical distance of data points from the mean. A larger standard deviation implies greater variability, while a smaller standard deviation implies less variability.
Interpreting Variance and Standard Deviation
The interpretation of variance and standard deviation depends heavily on the context of the data. There isn't a universally "good" or "bad" variance value. The key is to compare the variance or standard deviation to other relevant data or benchmarks.
For example:
- Comparing Investment Portfolios: A portfolio with a higher variance than another suggests higher risk, but potentially higher returns.
- Quality Control: A manufacturing process with a lower variance indicates higher consistency in producing items that meet specifications.
- Scientific Experiments: A smaller variance in experimental results indicates higher precision and reliability.
Remember that variance and standard deviation are descriptive statistics. They describe the characteristics of your specific dataset. They don’t offer insights into the cause of the variability or whether the variability is statistically significant.
Advanced Techniques and Considerations
- Sampling Variance: When calculating variance from a sample, we use a slightly modified formula to get an unbiased estimate of the population variance. This involves dividing by (n-1) instead of n, where n is the sample size.
- Weighted Variance: If some data points are more important than others, we can use weighted variance. This assigns different weights to data points based on their importance.
- Covariance and Correlation: Variance is a building block for understanding covariance and correlation, which describe the relationship between two or more variables.
Conclusion
Calculating and interpreting the variance of a probability distribution is a fundamental skill in statistics. Whether dealing with discrete or continuous data, understanding the formulas and their applications allows for a deeper understanding of data variability and uncertainty. Remember to consider the context of your data when interpreting variance and standard deviation, and don't hesitate to explore advanced techniques as your statistical needs become more complex. This comprehensive guide has equipped you with the knowledge to confidently tackle variance calculations in various scenarios, furthering your understanding of probability and statistics. By mastering these concepts, you'll be better prepared to analyze data effectively and make informed decisions based on your findings. Remember to always check your calculations and consider the limitations of your data when drawing conclusions.
Latest Posts
Latest Posts
-
What Type Of Cells Have The Most Mitochondria
Apr 27, 2025
-
Is N Or O More Electronegative
Apr 27, 2025
-
Acceleration Is Always In The Direction Of
Apr 27, 2025
-
What Prevents Backflow Of Blood During Circulation
Apr 27, 2025
-
The Backbone Of Dna Is Composed Of
Apr 27, 2025
Related Post
Thank you for visiting our website which covers about Find The Variance Of The Probability Distribution . We hope the information provided has been useful to you. Feel free to contact us if you have any questions or need further assistance. See you next time and don't miss to bookmark.