A Probability Distribution Is Also Called The Probability Function.
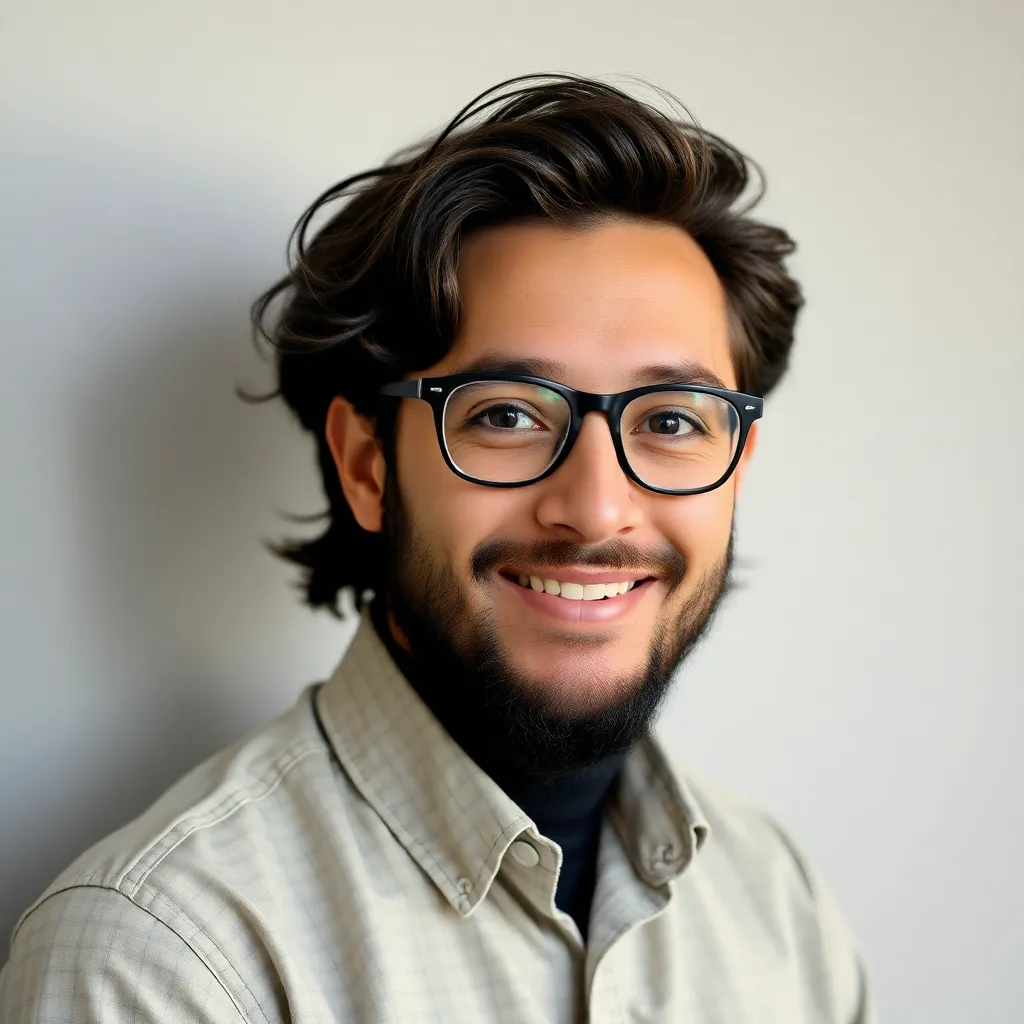
News Leon
Apr 17, 2025 · 7 min read

Table of Contents
A Probability Distribution is Also Called the Probability Function: A Deep Dive
A probability distribution, often interchangeably referred to as a probability function, is a fundamental concept in probability and statistics. It describes the likelihood of different outcomes or values for a random variable. Understanding probability distributions is crucial for a wide range of applications, from analyzing financial markets and predicting weather patterns to designing effective medical trials and understanding the spread of diseases. This article will delve deep into the concept, exploring its various types, properties, and applications.
What is a Probability Distribution?
In essence, a probability distribution mathematically describes the possible values a random variable can take and their associated probabilities. A random variable is a variable whose value is a numerical outcome of a random phenomenon. For instance, the outcome of rolling a die is a random variable, with possible values ranging from 1 to 6. The probability distribution would specify the probability of each outcome (assuming a fair die, each outcome has a probability of 1/6).
The term "probability function" is often used interchangeably with "probability distribution," particularly when dealing with discrete random variables. The function assigns a probability to each possible value of the random variable. While subtle differences exist in the formal mathematical definitions, in practical applications, the terms are frequently used synonymously.
Types of Probability Distributions
Probability distributions are broadly categorized into two main types:
1. Discrete Probability Distributions
Discrete probability distributions deal with random variables that can only take on a finite number of values or a countably infinite number of values. These values are typically integers. Examples include:
- Bernoulli Distribution: This describes the probability of success or failure in a single trial. Think of flipping a coin – the outcome is either heads (success) or tails (failure).
- Binomial Distribution: This models the probability of getting a certain number of successes in a fixed number of independent Bernoulli trials. For example, what's the probability of getting exactly 3 heads in 5 coin flips?
- Poisson Distribution: This describes the probability of a given number of events occurring in a fixed interval of time or space, given a known average rate of occurrence. This is useful for modeling events like the number of customers arriving at a store in an hour.
- Geometric Distribution: This distribution describes the probability of the number of trials needed to get the first success in a sequence of independent Bernoulli trials. For example, how many times do you need to roll a die before you get a 6?
- Negative Binomial Distribution: This is a generalization of the geometric distribution. It models the number of trials needed to achieve a specified number of successes.
The probability mass function (PMF) is used to define a discrete probability distribution. The PMF, often denoted as P(X=x), gives the probability that the random variable X takes on the specific value x.
2. Continuous Probability Distributions
Continuous probability distributions deal with random variables that can take on any value within a given range or interval. Unlike discrete distributions, the probability of a single specific value is zero. Instead, we talk about the probability of the variable falling within a certain interval. Examples include:
- Normal Distribution (Gaussian Distribution): This is arguably the most famous probability distribution. It's bell-shaped and symmetrical, with many real-world phenomena approximately following this distribution, like human height or IQ scores. It's characterized by its mean (μ) and standard deviation (σ).
- Uniform Distribution: This assigns equal probability to all values within a given range. Imagine choosing a random number between 0 and 1 – each number has an equal chance of being selected.
- Exponential Distribution: This distribution is often used to model the time until an event occurs, such as the lifespan of a component or the time between arrivals in a queue.
- Gamma Distribution: A versatile distribution that generalizes the exponential distribution and finds applications in various fields, including modeling waiting times and survival analysis.
- Beta Distribution: Used to model probabilities themselves, it's often employed in Bayesian statistics and modeling proportions.
- Chi-Squared Distribution: Frequently used in hypothesis testing and related statistical applications.
- t-Distribution: Similar to the normal distribution but with heavier tails; often used when dealing with smaller sample sizes.
- F-Distribution: Employed in analysis of variance (ANOVA) and comparing variances between different groups.
The probability density function (PDF) is used to define a continuous probability distribution. The PDF, often denoted as f(x), doesn't directly give the probability of a specific value but rather the relative likelihood of the variable falling within a small interval around that value. The probability of the variable falling within a specific range is found by integrating the PDF over that range.
Properties of Probability Distributions
Regardless of whether a distribution is discrete or continuous, several key properties define it:
- Mean (Expected Value): The average value of the random variable. This represents the central tendency of the distribution.
- Variance: A measure of how spread out the distribution is. A high variance indicates greater dispersion, while a low variance indicates values clustered closely around the mean.
- Standard Deviation: The square root of the variance. It's expressed in the same units as the random variable, making it easier to interpret than the variance.
- Skewness: A measure of the asymmetry of the distribution. A positive skew indicates a longer tail on the right, while a negative skew indicates a longer tail on the left.
- Kurtosis: A measure of the "tailedness" of the probability distribution. It describes the sharpness of the peak and the heaviness of the tails relative to a normal distribution.
Applications of Probability Distributions
Probability distributions have a vast array of applications across various disciplines:
- Finance: Modeling stock prices, predicting risk, and valuing financial assets. The normal distribution and its variations are commonly used in financial modeling, although other distributions are also relevant.
- Insurance: Assessing risk, calculating premiums, and managing reserves. Actuarial science relies heavily on probability distributions to model claims and losses.
- Engineering: Reliability analysis, quality control, and designing robust systems. Understanding the distribution of component failures is crucial in engineering design.
- Medicine: Designing clinical trials, analyzing medical data, and predicting disease outbreaks. Probability distributions help determine sample sizes, assess treatment efficacy, and model disease progression.
- Machine Learning: Probability distributions are fundamental to many machine learning algorithms, particularly Bayesian methods. They are used to model data, make predictions, and estimate parameters.
- Physics: Modeling physical phenomena, analyzing experimental data, and making predictions. Probability distributions are used in quantum mechanics, statistical mechanics, and other areas of physics.
- Meteorology: Predicting weather patterns, analyzing climate data, and assessing extreme weather events.
Choosing the Right Probability Distribution
Selecting the appropriate probability distribution for a given problem is crucial for accurate analysis and reliable predictions. The choice depends on several factors, including:
- The nature of the data: Is the data discrete or continuous?
- The shape of the data: Is the data symmetric, skewed, or otherwise?
- The underlying process: What is the mechanism generating the data?
- Prior knowledge or assumptions: Are there any prior beliefs about the distribution?
Often, data visualization and statistical tests help in determining which distribution best fits the observed data. Tools like histograms, Q-Q plots, and goodness-of-fit tests can assist in this process.
Conclusion
Probability distributions are a cornerstone of probability and statistics, providing a powerful framework for modeling and analyzing random phenomena. Understanding the different types of distributions, their properties, and their applications is essential across a broad spectrum of fields. The seemingly simple concept of assigning probabilities to outcomes has far-reaching consequences, impacting our ability to make informed decisions and predictions in the face of uncertainty. While the terms "probability distribution" and "probability function" are frequently used interchangeably, appreciating the nuances between them strengthens the foundational understanding of this crucial statistical concept. By mastering the principles of probability distributions, one can unlock valuable insights and gain a more comprehensive grasp of the world around us.
Latest Posts
Related Post
Thank you for visiting our website which covers about A Probability Distribution Is Also Called The Probability Function. . We hope the information provided has been useful to you. Feel free to contact us if you have any questions or need further assistance. See you next time and don't miss to bookmark.